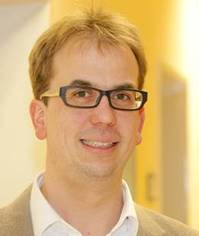
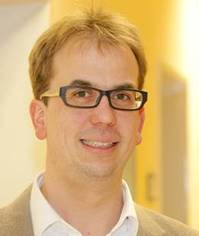
Helge Frieling
Helge is deputy Director of a psychiatric university hospital and full professor of molecular psychiatry with 10+ years of experience in clinical, strategic and scientific leadership. He established translational science with international and national collaborations by building up a Molecular Biology Laboratory and the Research Core Unit Genomics. Helge is among the leading German researchers in psychiatric epigenetics with 50+ original publications dealing with DNA methylation. He's a dedicated teacher for medical students, nurses, Ph.D. candidates and early-career researchers with 15+ years of experience in lectures, lab-classes, and bed-side teaching. Helge is a clinical psychiatrist devoted to the advanced and personalized treatment of patients.
Big data and mental health - moving forward to precision psychiatry
One of the major obstacles in today’s psychiatric research is the obvious mismatch between the diagnostic categories stemming mainly from the end of the 19th century and modern neurobiological concepts of normal and disrupted brain function, leading to a lag in the development of new and more effective therapies/therapeutics. One main research goal of my group is the use of (epi-)genetic markers to detect and categorize biologically distinct sub-groups of psychiatric disorders, using therapy response as a primary phenotype. We were able to establish DNA methylation markers indicating an elevated risk for non-response to standard mono-aminergic antidepressants (EU and US patent granted) or for non-response under electro-convulsive therapy (ECT). Further DNA methylation markers that predict positive response to ECT and specific psychotherapeutic treatments are currently being tested. Large data sets derived from the different branches of basic or clinical research contain a wealth of information. To access and implement this information, new methods of data analysis are needed. Pattern recognition based on artificial intelligence/neuronal networks is a feasible approach to tackle these research questions. During recent years, we have gained expertise in the use of these “big-data” methods for the integrative analysis of molecular and clinical data. The use of self-learning algorithms not only helps to discover new and unexpected relationships between molecular and clinical data but also fosters the development of diagnostic and treatment algorithms in an iterative and evolutionary way (plan-do-check-act (PDCA)-cycle integrating patient’s care and research goals), paving the way for precision psychiatry.