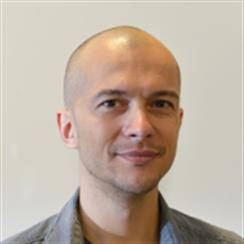
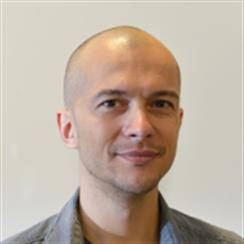
Prof. Dr. Robert Hoehndorf
Robert Hoehndorf is an Associate Professor in Computer Science at King Abdullah University of Science and Technology (KAUST) in Thuwal. Prior to joining KAUST, Robert had research positions at Aberystwyth University, the University of Cambridge, the European Bioinformatics Institute, and the Max Planck Institute for Evolutionary Anthropology. His research focuses on the development and application of knowledge-based algorithms in biology and biomedicine.
Tutorial I: Semantic similarity and machine learning with biomedical ontologies
Ontologies are widely used to characterize biomedical data and ensure interoperability between different databases. I will provide an introduction into methods that use biomedical ontologies in machine learning models. I will first introduce semantic similarity measures that rely on axioms in ontologies to compare domain entities. From semantic similarity, I will develop and discuss unsupervised machine learning methods that "embed" ontology axioms in vector spaces, and show how they can be used to capture semantic similarity. Through a set of executable notebooks, we will then apply these methods to the task of diagnosing rare disease patients based on machine learning over phenotype ontologies.
Tutorial II: Semantic similarity and machine learning with biomedical ontologies
Ontologies are widely used to characterize biomedical data and ensure interoperability between different databases. I will provide an introduction into methods that use biomedical ontologies in machine learning models. I will first introduce semantic similarity measures that rely on axioms in ontologies to compare domain entities. From semantic similarity, I will develop and discuss unsupervised machine learning methods that "embed" ontology axioms in vector spaces, and show how they can be used to capture semantic similarity. Through a set of executable notebooks, we will then apply these methods to the task of diagnosing rare disease patients based on machine learning over phenotype ontologies.
Machine learning with biomedical ontologies for precision health
The life sciences have invested significant resources in the development and application of semantic technologies to make research data accessible and interlinked, and to enable the integration and analysis of data. Utilizing the semantics associated with research data in data analysis approaches is often challenging. Now, novel methods are becoming available that combine symbolic methods and statistical methods in Artificial Intelligence. In my talk, I will show how to incorporate biological background knowledge in machine learning models for identification of gene-disease associations, genomic variants that are causative for heritable disorders, and to predict protein functions. The methods I describe are generic and can be applied in other domains in which biomedical ontologies and structured knowledge bases exist.