
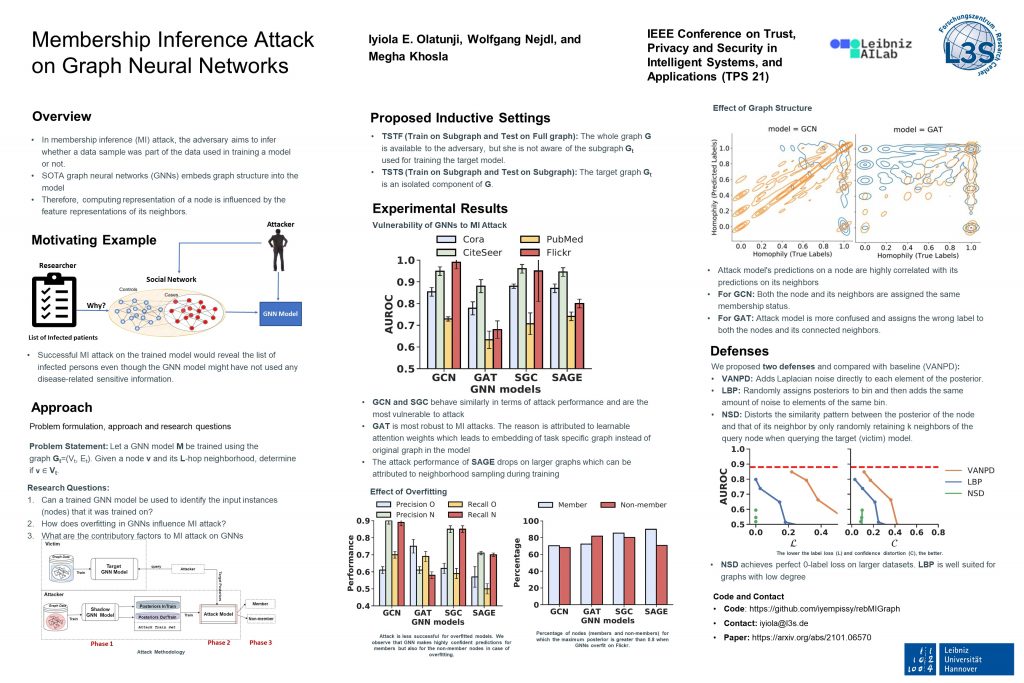
- Dockhorn, A., Eberhardinger, M., Loiacono, D., Liebana, D. P., and Veltkamp, R. (2023)Pokegen, Human-Game AI Interaction (Dagstuhl Seminar 22251) 12, 39–42.
@article{DocEbe2023a,
author = {Dockhorn, Alexander and Eberhardinger, Manuel and Loiacono, Daniele and Liebana, Diego Perez and Veltkamp, Remco},
journal = {Human-Game AI Interaction (Dagstuhl Seminar 22251)},
keywords = {Pokegen},
month = {01},
number = 6,
pages = {39-42},
title = {Pokegen},
volume = 12,
year = 2023
}%0 Journal Article
%1 DocEbe2023a
%A Dockhorn, Alexander
%A Eberhardinger, Manuel
%A Loiacono, Daniele
%A Liebana, Diego Perez
%A Veltkamp, Remco
%D 2023
%J Human-Game AI Interaction (Dagstuhl Seminar 22251)
%N 6
%P 39-42
%R 10.4230/DagRep.12.6.28
%T Pokegen
%V 12 - Nandy, A., Kapadnis, M. N., Goyal, P., and Ganguly, N. (2023)CLMSM: A Multi-Task Learning Framework for Pre-training on Procedural Text. In The 2023 Conference on Empirical Methods in Natural Language Processing.
@inproceedings{nandy2023textbfemphclmsm,
author = {Nandy, Abhilash and Kapadnis, Manav Nitin and Goyal, Pawan and Ganguly, Niloy},
booktitle = {The 2023 Conference on Empirical Methods in Natural Language Processing},
keywords = {pretraining},
title = {CLMSM: A Multi-Task Learning Framework for Pre-training on Procedural Text},
year = 2023
}%0 Conference Paper
%1 nandy2023textbfemphclmsm
%A Nandy, Abhilash
%A Kapadnis, Manav Nitin
%A Goyal, Pawan
%A Ganguly, Niloy
%B The 2023 Conference on Empirical Methods in Natural Language Processing
%D 2023
%T CLMSM: A Multi-Task Learning Framework for Pre-training on Procedural Text
%U https://openreview.net/forum?id=SP8zIwanHD - Auer, S., Barone, D. A. C., Bartz, C., Cortes, E. G., Jaradeh, M. Y., Karras, O., Koubarakis, M., Mouromtsev, D., Pliukhin, D., Radyush, D., Shilin, I., Stocker, M., and Tsalapati, E. (2023, March)SciQA benchmark: Dataset and {RDF} dump (Version 5), Zenodo.
@misc{DBLP:data/10/AuerBBCJKKMPRSST23a,
author = {Auer, S{{ö}}ren and Barone, Dante A. C. and Bartz, Cassiano and Cortes, Eduardo G. and Jaradeh, Mohamad Yaser and Karras, Oliver and Koubarakis, Manolis and Mouromtsev, Dmitry and Pliukhin, Dmitrii and Radyush, Daniil and Shilin, Ivan and Stocker, Markus and Tsalapati, Eleni},
howpublished = {\url{https://doi.org/10.5281/zenodo.7727922}},
keywords = {leibnizailab},
month = {03},
note = {Accessed on YYYY-MM-DD.},
publisher = {Zenodo},
title = {SciQA benchmark: Dataset and {RDF} dump (Version 5)},
year = 2023
}%0 Generic
%1 DBLP:data/10/AuerBBCJKKMPRSST23a
%A Auer, S{{ö}}ren
%A Barone, Dante A. C.
%A Bartz, Cassiano
%A Cortes, Eduardo G.
%A Jaradeh, Mohamad Yaser
%A Karras, Oliver
%A Koubarakis, Manolis
%A Mouromtsev, Dmitry
%A Pliukhin, Dmitrii
%A Radyush, Daniil
%A Shilin, Ivan
%A Stocker, Markus
%A Tsalapati, Eleni
%D 2023
%I Zenodo
%R 10.5281/ZENODO.7727922
%T SciQA benchmark: Dataset and {RDF} dump (Version 5)
%U https://doi.org/10.5281/zenodo.7727922 - Kuhnke, F., and Ostermann, J. (2023)Domain Adaptation for Head Pose Estimation Using Relative Pose Consistency, IEEE Transactions on Biometrics, Behavior, and Identity Science.
@article{KuhOst2023,
author = {Kuhnke, Felix and Ostermann, J{ö}rn},
journal = {IEEE Transactions on Biometrics, Behavior, and Identity Science},
keywords = {Adaptation},
title = {Domain Adaptation for Head Pose Estimation Using Relative Pose Consistency},
year = 2023
}%0 Journal Article
%1 KuhOst2023
%A Kuhnke, Felix
%A Ostermann, J{ö}rn
%D 2023
%J IEEE Transactions on Biometrics, Behavior, and Identity Science
%R 10.1109/TBIOM.2023.3237039
%T Domain Adaptation for Head Pose Estimation Using Relative Pose Consistency - Poker, Y., von Hardenberg, S., Hofmann, W., Tang, M., Baumann, U., Schwerk, N., Wetzke, M., Lindenthal, V., Auber, B., Schlegelberger, B., Ott, H., von Bismarck, P., Viemann, D., Dressler, F., Klemann, C., and Bergmann, A. K. (2023)Systematic genetic analysis of pediatric patients with autoinflammatory diseases, Frontiers in Genetics, Frontiers Media {SA} 14.
@article{Poker_2023,
author = {Poker, Yvonne and von Hardenberg, Sandra and Hofmann, Winfried and Tang, Ming and Baumann, Ulrich and Schwerk, Nicolaus and Wetzke, Martin and Lindenthal, Viola and Auber, Bernd and Schlegelberger, Brigitte and Ott, Hagen and von Bismarck, Philipp and Viemann, Dorothee and Dressler, Frank and Klemann, Christian and Bergmann, Anke Katharina},
journal = {Frontiers in Genetics},
keywords = {l3s},
month = {01},
publisher = {Frontiers Media {SA}},
title = {Systematic genetic analysis of pediatric patients with autoinflammatory diseases},
volume = 14,
year = 2023
}%0 Journal Article
%1 Poker_2023
%A Poker, Yvonne
%A von Hardenberg, Sandra
%A Hofmann, Winfried
%A Tang, Ming
%A Baumann, Ulrich
%A Schwerk, Nicolaus
%A Wetzke, Martin
%A Lindenthal, Viola
%A Auber, Bernd
%A Schlegelberger, Brigitte
%A Ott, Hagen
%A von Bismarck, Philipp
%A Viemann, Dorothee
%A Dressler, Frank
%A Klemann, Christian
%A Bergmann, Anke Katharina
%D 2023
%I Frontiers Media {SA}
%J Frontiers in Genetics
%R 10.3389/fgene.2023.1065907
%T Systematic genetic analysis of pediatric patients with autoinflammatory diseases
%U https://doi.org/10.3389%2Ffgene.2023.1065907
%V 14 - Adhisantoso, Y. G., and Voges, J. (2023)Cross-check of M62859 Results on Updated CE Results for Annotation Data Indexing Using B-Tree, ISO/IEC JTC 1/SC 29/WG 8.
@article{AdhVog2023a,
author = {Adhisantoso, Yeremia Gunawan and Voges, Jan},
journal = {ISO/IEC JTC 1/SC 29/WG 8},
keywords = {M62859},
month = {04},
title = {Cross-check of M62859 Results on Updated CE Results for Annotation Data Indexing Using B-Tree},
year = 2023
}%0 Journal Article
%1 AdhVog2023a
%A Adhisantoso, Yeremia Gunawan
%A Voges, Jan
%D 2023
%J ISO/IEC JTC 1/SC 29/WG 8
%T Cross-check of M62859 Results on Updated CE Results for Annotation Data Indexing Using B-Tree - Kaiser, T., Reinders, C., and Rosenhahn, B. (2023)Compensation Learning in Semantic Segmentation. In Computer Vision and Pattern Recognition Workshops (CVPRW).
@inproceedings{KaiRei2023a,
author = {Kaiser, Timo and Reinders, Christoph and Rosenhahn, Bodo},
booktitle = {Computer Vision and Pattern Recognition Workshops (CVPRW)},
keywords = {Segmention},
month = {06},
title = {Compensation Learning in Semantic Segmentation},
year = 2023
}%0 Conference Paper
%1 KaiRei2023a
%A Kaiser, Timo
%A Reinders, Christoph
%A Rosenhahn, Bodo
%B Computer Vision and Pattern Recognition Workshops (CVPRW)
%D 2023
%T Compensation Learning in Semantic Segmentation - Roy, S., Wallat, J., Sundaram, S. S., Nejdl, W., and Ganguly, N. (2023)GENEMASK: Fast Pretraining of Gene Sequences to Enable Few-Shot Learning. In Frontiers in Artificial Intelligence and Applications, pp. 2002–2009.Large-scale language models such as DNABert and LOGO aim to learn optimal gene representations and are trained on the entire Human Reference Genome. However, standard tokenization schemes involve a simple sliding window of tokens like k-mers that do not leverage any gene-based semantics and thus may lead to (trivial) masking of easily predictable sequences, and subsequently inefficient Masked Language Modeling (MLM) training. Therefore, we propose a novel masking algorithm, GENEMASK, for MLM training of gene sequences, where we randomly identify positions in a gene sequence as mask centers and locally select the span around the mask center with the highest Normalized Pointwise Mutual Information (NPMI) to mask. We observe that in the absence of human-understandable semantics in the genomics domain (in contrast, semantic units like words and phrases are inherently available in NLP), GENEMASK-based models substantially outperform the SOTA models (DNABert and LOGO) over four benchmark gene sequence classification datasets in five few-shot settings (10 to 1000-shot). More significantly, the GENEMASK-based DNABert model is trained for less than one-tenth of the number of epochs of the original SOTA model. We also observe a strong correlation between top-ranked PMI tokens and conserved DNA sequence motifs, which may indicate the incorporation of latent genomic information. The codes (including trained models) and datasets are made publicly available at unmapped: uri https://github.com/roysoumya/GeneMask.
@inproceedings{noauthororeditor,
abstract = {Large-scale language models such as DNABert and LOGO aim to learn optimal gene representations and are trained on the entire Human Reference Genome. However, standard tokenization schemes involve a simple sliding window of tokens like k-mers that do not leverage any gene-based semantics and thus may lead to (trivial) masking of easily predictable sequences, and subsequently inefficient Masked Language Modeling (MLM) training. Therefore, we propose a novel masking algorithm, GENEMASK, for MLM training of gene sequences, where we randomly identify positions in a gene sequence as mask centers and locally select the span around the mask center with the highest Normalized Pointwise Mutual Information (NPMI) to mask. We observe that in the absence of human-understandable semantics in the genomics domain (in contrast, semantic units like words and phrases are inherently available in NLP), GENEMASK-based models substantially outperform the SOTA models (DNABert and LOGO) over four benchmark gene sequence classification datasets in five few-shot settings (10 to 1000-shot). More significantly, the GENEMASK-based DNABert model is trained for less than one-tenth of the number of epochs of the original SOTA model. We also observe a strong correlation between top-ranked PMI tokens and conserved DNA sequence motifs, which may indicate the incorporation of latent genomic information. The codes (including trained models) and datasets are made publicly available at unmapped: uri https://github.com/roysoumya/GeneMask.},
author = {Roy, Soumyadeep and Wallat, Jonas and Sundaram, Sowmya S and Nejdl, Wolfgang and Ganguly, Niloy},
keywords = {l3s},
pages = {2002-2009},
series = {Frontiers in Artificial Intelligence and Applications},
title = {GENEMASK: Fast Pretraining of Gene Sequences to Enable Few-Shot Learning},
volume = 372,
year = 2023
}%0 Conference Paper
%1 noauthororeditor
%A Roy, Soumyadeep
%A Wallat, Jonas
%A Sundaram, Sowmya S
%A Nejdl, Wolfgang
%A Ganguly, Niloy
%B Frontiers in Artificial Intelligence and Applications
%D 2023
%P 2002-2009
%R 10.3233/FAIA230492
%T GENEMASK: Fast Pretraining of Gene Sequences to Enable Few-Shot Learning
%V 372
%X Large-scale language models such as DNABert and LOGO aim to learn optimal gene representations and are trained on the entire Human Reference Genome. However, standard tokenization schemes involve a simple sliding window of tokens like k-mers that do not leverage any gene-based semantics and thus may lead to (trivial) masking of easily predictable sequences, and subsequently inefficient Masked Language Modeling (MLM) training. Therefore, we propose a novel masking algorithm, GENEMASK, for MLM training of gene sequences, where we randomly identify positions in a gene sequence as mask centers and locally select the span around the mask center with the highest Normalized Pointwise Mutual Information (NPMI) to mask. We observe that in the absence of human-understandable semantics in the genomics domain (in contrast, semantic units like words and phrases are inherently available in NLP), GENEMASK-based models substantially outperform the SOTA models (DNABert and LOGO) over four benchmark gene sequence classification datasets in five few-shot settings (10 to 1000-shot). More significantly, the GENEMASK-based DNABert model is trained for less than one-tenth of the number of epochs of the original SOTA model. We also observe a strong correlation between top-ranked PMI tokens and conserved DNA sequence motifs, which may indicate the incorporation of latent genomic information. The codes (including trained models) and datasets are made publicly available at unmapped: uri https://github.com/roysoumya/GeneMask.
%@ 978-1-64368-437-6 - Awiszus, M., Dockhorn, A., Hoover, A. K., Liapis, A., Lucas, S. M., Eladhari, M. P., Schrum, J., and Volz, V. (2023)Language Models for Procedural Content Generation, Human-Game AI Interaction (Dagstuhl Seminar 22251) 12, 34–37.
@article{AwiDoc2023,
author = {Awiszus, Maren and Dockhorn, Alexander and Hoover, Amy K. and Liapis, Antonios and Lucas, Simon M. and Eladhari, Mirjam Palosaari and Schrum, Jacob and Volz, Vanessa},
journal = {Human-Game AI Interaction (Dagstuhl Seminar 22251)},
keywords = {Language},
month = {01},
number = 6,
pages = {34-37},
title = {Language Models for Procedural Content Generation},
volume = 12,
year = 2023
}%0 Journal Article
%1 AwiDoc2023
%A Awiszus, Maren
%A Dockhorn, Alexander
%A Hoover, Amy K.
%A Liapis, Antonios
%A Lucas, Simon M.
%A Eladhari, Mirjam Palosaari
%A Schrum, Jacob
%A Volz, Vanessa
%D 2023
%J Human-Game AI Interaction (Dagstuhl Seminar 22251)
%N 6
%P 34-37
%R 10.4230/DagRep.12.6.28
%T Language Models for Procedural Content Generation
%V 12 - Lee, C.-S., Wang, M.-H., Chen, C.-Y., Yang, F.-J., and Dockhorn, A. (2023)Genetic Assessment Agent for High-School Student and Machine Co-Learning Model Construction on Computational Intelligence Experience. In 2023 IEEE Congress on Evolutionary Computation, pp. 1–8.
@inproceedings{LeeWan2023,
author = {Lee, Chang-Shing and Wang, Mei-Hui and Chen, Chih-Yu and Yang, Fu-Jie and Dockhorn, Alexander},
booktitle = {2023 IEEE Congress on Evolutionary Computation},
keywords = {Student},
note = {(to be published)},
pages = {1-8},
title = {Genetic Assessment Agent for High-School Student and Machine Co-Learning Model Construction on Computational Intelligence Experience},
year = 2023
}%0 Conference Paper
%1 LeeWan2023
%A Lee, Chang-Shing
%A Wang, Mei-Hui
%A Chen, Chih-Yu
%A Yang, Fu-Jie
%A Dockhorn, Alexander
%B 2023 IEEE Congress on Evolutionary Computation
%D 2023
%P 1-8
%T Genetic Assessment Agent for High-School Student and Machine Co-Learning Model Construction on Computational Intelligence Experience - Stahl, M., Spliethöver, M., and Wachsmuth, H. (2022)To Prefer or to Choose? Generating Agency and Power Counterfactuals Jointly for Gender Bias Mitigation. In Proceedings of the Fifth Workshop on Natural Language Processing and Computational Social Science.
@inproceedings{Stahl_Spliethöver_Wachsmuth,
author = {Stahl, Maja and Spliethöver, Maximilian and Wachsmuth, Henning},
booktitle = {Proceedings of the Fifth Workshop on Natural Language Processing and Computational Social Science},
keywords = {leibnizailab},
title = {To Prefer or to Choose? Generating Agency and Power Counterfactuals Jointly for Gender Bias Mitigation},
year = 2022
}%0 Conference Paper
%1 Stahl_Spliethöver_Wachsmuth
%A Stahl, Maja
%A Spliethöver, Maximilian
%A Wachsmuth, Henning
%B Proceedings of the Fifth Workshop on Natural Language Processing and Computational Social Science
%D 2022
%T To Prefer or to Choose? Generating Agency and Power Counterfactuals Jointly for Gender Bias Mitigation - Schier, M., Reinders, C., and Rosenhahn, B. (2022)Constrained Mean Shift Clustering. In Proceedings of the 2022 SIAM International Conference on Data Mining (SDM).
@inproceedings{SchRei2022a,
author = {Schier, Maximilian and Reinders, Christoph and Rosenhahn, Bodo},
booktitle = {Proceedings of the 2022 SIAM International Conference on Data Mining (SDM)},
keywords = {Clustering},
month = {04},
title = {Constrained Mean Shift Clustering},
year = 2022
}%0 Conference Paper
%1 SchRei2022a
%A Schier, Maximilian
%A Reinders, Christoph
%A Rosenhahn, Bodo
%B Proceedings of the 2022 SIAM International Conference on Data Mining (SDM)
%D 2022
%T Constrained Mean Shift Clustering
%U https://github.com/m-schier/cms - Mast, M., Marschollek, M., Jack, T., Wulff, A., and Elise Study, G. (2022)Developing a Data Driven Approach for Early Detection of SIRS in Pediatric Intensive Care Using Automatically Labeled Training Data, Stud Health Technol Inform 289, 228–231.
@article{RN11,
author = {Mast, M. and Marschollek, M. and Jack, T. and Wulff, A. and Elise Study, Group},
journal = {Stud Health Technol Inform},
keywords = {l3s},
pages = {228-231},
title = {Developing a Data Driven Approach for Early Detection of SIRS in Pediatric Intensive Care Using Automatically Labeled Training Data},
type = {Journal Article},
volume = 289,
year = 2022
}%0 Journal Article
%1 RN11
%A Mast, M.
%A Marschollek, M.
%A Jack, T.
%A Wulff, A.
%A Elise Study, Group
%D 2022
%J Stud Health Technol Inform
%P 228-231
%R 10.3233/SHTI210901
%T Developing a Data Driven Approach for Early Detection of SIRS in Pediatric Intensive Care Using Automatically Labeled Training Data
%U https://www.ncbi.nlm.nih.gov/pubmed/35062134
%V 289 - Bondarenko, A., Fr{ö}be, M., Kiesel, J., Syed, S., Gurcke, T., Beloucif, M., Panchenko, A., Biemann, C., Stein, B., Wachsmuth, H., Potthast, M., and Hagen, M. (2022)Overview of Touch{é} 2022: Argument Retrieval, CEUR Workshop Proceedings, CEUR WS 3180, 2867–2903.This paper is a report on the third year of the Touch{é} lab on argument retrieval hosted at CLEF 2022. With the goal of supporting and promoting the research and development of new technologies for argument mining and argument analysis, we have organized three shared tasks: (a) argument retrieval for controversial topics, where the task is to find sentences that reflect the gist of arguments from online debates, (b) argument retrieval for comparative issues, where the task is to find argumentative passages from web documents that help in making a comparative decision, and (c) image retrieval for arguments, where the task is to find images that show support for or opposition to a particular stance.
@article{c1861a701a4f42559399a794a05b1b29,
abstract = {This paper is a report on the third year of the Touch{é} lab on argument retrieval hosted at CLEF 2022. With the goal of supporting and promoting the research and development of new technologies for argument mining and argument analysis, we have organized three shared tasks: (a) argument retrieval for controversial topics, where the task is to find sentences that reflect the gist of arguments from online debates, (b) argument retrieval for comparative issues, where the task is to find argumentative passages from web documents that help in making a comparative decision, and (c) image retrieval for arguments, where the task is to find images that show support for or opposition to a particular stance.},
author = {Bondarenko, Alexander and Fr{ö}be, Maik and Kiesel, Johannes and Syed, Shahbaz and Gurcke, Timon and Beloucif, Meriem and Panchenko, Alexander and Biemann, Chris and Stein, Benno and Wachsmuth, Henning and Potthast, Martin and Hagen, Matthias},
journal = {CEUR Workshop Proceedings},
keywords = {leibnizailab},
note = {Funding Information: This work was partially supported by the Deutsche Forschungsgemeinschaft (DFG) through the projects “ACQuA 2.0” (Answering Comparative Questions with Arguments; project number 376430233) and “OASiS” (Objective Argument Summarization in Search; project number 455913891) as part of the priority program “RATIO: Robust Argumentation Machines” (SPP 1999), and the German Ministry for Science and Education (BMBF) through the project “SharKI” (Shared Tasks as an Innovative Approach to Implement AI and Big Data-based Applications within Universities; grant FKZ 16DHB4021). We are also grateful to Jan Heinrich Reimer for developing the TARGER Python library and Erik Reuter for expanding a document collection for Task 2 with docT5query.; 2022 Conference and Labs of the Evaluation Forum, CLEF 2022 ; Conference date: 05-09-2022 Through 08-09-2022},
pages = {2867--2903},
publisher = {CEUR WS},
title = {Overview of Touch{é} 2022: Argument Retrieval},
volume = 3180,
year = 2022
}%0 Journal Article
%1 c1861a701a4f42559399a794a05b1b29
%A Bondarenko, Alexander
%A Fr{ö}be, Maik
%A Kiesel, Johannes
%A Syed, Shahbaz
%A Gurcke, Timon
%A Beloucif, Meriem
%A Panchenko, Alexander
%A Biemann, Chris
%A Stein, Benno
%A Wachsmuth, Henning
%A Potthast, Martin
%A Hagen, Matthias
%D 2022
%I CEUR WS
%J CEUR Workshop Proceedings
%P 2867--2903
%T Overview of Touch{é} 2022: Argument Retrieval
%V 3180
%X This paper is a report on the third year of the Touch{é} lab on argument retrieval hosted at CLEF 2022. With the goal of supporting and promoting the research and development of new technologies for argument mining and argument analysis, we have organized three shared tasks: (a) argument retrieval for controversial topics, where the task is to find sentences that reflect the gist of arguments from online debates, (b) argument retrieval for comparative issues, where the task is to find argumentative passages from web documents that help in making a comparative decision, and (c) image retrieval for arguments, where the task is to find images that show support for or opposition to a particular stance. - Sass, R., Bergman, E., Biedenkapp, A., Hutter, F., and Lindauer, M. (2022)DeepCAVE: An Interactive Analysis Tool for Automated Machine Learning. In ICML Workshop on Adaptive Experimental Design and Active Learning in the Real World (ReALML), arXiv.
@inproceedings{https://doi.org/10.48550/arxiv.2206.03493,
author = {Sass, René and Bergman, Eddie and Biedenkapp, André and Hutter, Frank and Lindauer, Marius},
booktitle = {ICML Workshop on Adaptive Experimental Design and Active Learning in the Real World (ReALML)},
keywords = {leibnizailab},
publisher = {arXiv},
title = {DeepCAVE: An Interactive Analysis Tool for Automated Machine Learning},
year = 2022
}%0 Conference Paper
%1 https://doi.org/10.48550/arxiv.2206.03493
%A Sass, René
%A Bergman, Eddie
%A Biedenkapp, André
%A Hutter, Frank
%A Lindauer, Marius
%B ICML Workshop on Adaptive Experimental Design and Active Learning in the Real World (ReALML)
%D 2022
%I arXiv
%R 10.48550/ARXIV.2206.03493
%T DeepCAVE: An Interactive Analysis Tool for Automated Machine Learning
%U https://arxiv.org/abs/2206.03493 - Xu, L., Hurtado-Grueso, J., Jeurissen, D., Liebana, D. P., and Dockhorn, A. (2022)Elastic Monte Carlo Tree Search State Abstraction for Strategy Game Playing. In 2022 IEEE Conference on Games (CoG).
@inproceedings{XuHur2022,
author = {Xu, Linjie and Hurtado-Grueso, Jorge and Jeurissen, Dominic and Liebana, Diego Perez and Dockhorn, Alexander},
booktitle = {2022 IEEE Conference on Games (CoG)},
keywords = {Monte},
title = {Elastic Monte Carlo Tree Search State Abstraction for Strategy Game Playing},
year = 2022
}%0 Conference Paper
%1 XuHur2022
%A Xu, Linjie
%A Hurtado-Grueso, Jorge
%A Jeurissen, Dominic
%A Liebana, Diego Perez
%A Dockhorn, Alexander
%B 2022 IEEE Conference on Games (CoG)
%D 2022
%T Elastic Monte Carlo Tree Search State Abstraction for Strategy Game Playing
%U https://arxiv.org/abs/2205.15126 - Dockhorn, A., Kirst, M., Mostaghim, S., Wieczorek, M., and Zille, H. (2022)Evolutionary Algorithm for Parameter Optimization of Context Steering Agents, IEEE Transactions on Games 1–12.
@article{DocKir2022,
author = {Dockhorn, Alexander and Kirst, Martin and Mostaghim, Sanaz and Wieczorek, Martin and Zille, Heiner},
journal = {IEEE Transactions on Games},
keywords = {Algorithm},
pages = {1-12},
title = {Evolutionary Algorithm for Parameter Optimization of Context Steering Agents},
year = 2022
}%0 Journal Article
%1 DocKir2022
%A Dockhorn, Alexander
%A Kirst, Martin
%A Mostaghim, Sanaz
%A Wieczorek, Martin
%A Zille, Heiner
%D 2022
%J IEEE Transactions on Games
%P 1-12
%R 10.1109/TG.2022.3157247
%T Evolutionary Algorithm for Parameter Optimization of Context Steering Agents
%U https://ieeexplore.ieee.org/document/9729529 - Dockhorn, A., and Kruse, R. (2022)Balancing Exploration and Exploitation in Forward Model Learning, Advances in Intelligent Systems Research and Innovation 1–19.
@article{DocKru2022,
author = {Dockhorn, Alexander and Kruse, Rudolf},
journal = {Advances in Intelligent Systems Research and Innovation},
keywords = {Exploration},
pages = {1--19},
title = {Balancing Exploration and Exploitation in Forward Model Learning},
year = 2022
}%0 Journal Article
%1 DocKru2022
%A Dockhorn, Alexander
%A Kruse, Rudolf
%D 2022
%J Advances in Intelligent Systems Research and Innovation
%P 1--19
%R 10.1007/978-3-030-78124-8_1
%T Balancing Exploration and Exploitation in Forward Model Learning
%U https://doi.org/10.1007/978-3-030-78124-8_1
%@ 978-3-030-78124-8 - Moosbauer, J., Casalicchio, G., Lindauer, M., and Bischl, B. (2022)Improving Accuracy of Interpretability Measures in Hyperparameter Optimization via Bayesian Algorithm Execution, arXiv.
@misc{https://doi.org/10.48550/arxiv.2206.05447,
author = {Moosbauer, Julia and Casalicchio, Giuseppe and Lindauer, Marius and Bischl, Bernd},
keywords = {leibnizailab},
publisher = {arXiv},
title = {Improving Accuracy of Interpretability Measures in Hyperparameter Optimization via Bayesian Algorithm Execution},
year = 2022
}%0 Generic
%1 https://doi.org/10.48550/arxiv.2206.05447
%A Moosbauer, Julia
%A Casalicchio, Giuseppe
%A Lindauer, Marius
%A Bischl, Bernd
%D 2022
%I arXiv
%R 10.48550/ARXIV.2206.05447
%T Improving Accuracy of Interpretability Measures in Hyperparameter Optimization via Bayesian Algorithm Execution
%U https://arxiv.org/abs/2206.05447 - Alshomary, M., and Stahl, M. (2022)Argument Novelty and Validity Assessment via Multitask and Transfer Learning. In Proceedings of the 9th Workshop on Argument Mining, pp. 111–114, International Conference on Computational Linguistics.
@inproceedings{Alshomary_Stahl_2022,
author = {Alshomary, Milad and Stahl, Maja},
booktitle = {Proceedings of the 9th Workshop on Argument Mining},
keywords = {leibnizailab},
pages = {111–114},
publisher = {International Conference on Computational Linguistics},
title = {Argument Novelty and Validity Assessment via Multitask and Transfer Learning},
year = 2022
}%0 Conference Paper
%1 Alshomary_Stahl_2022
%A Alshomary, Milad
%A Stahl, Maja
%B Proceedings of the 9th Workshop on Argument Mining
%D 2022
%I International Conference on Computational Linguistics
%P 111–114
%T Argument Novelty and Validity Assessment via Multitask and Transfer Learning - Hinrichs, R., Liang, K., Lu, Z., and Ostermann, J. (2022)Improved Compression of Artificial Neural Networks through Curvature-Aware Training. In Proceedings of the IEEE World Congress on Computational Intelligence.
@inproceedings{HinLia2022,
author = {Hinrichs, Reemt and Liang, Kai and Lu, Ze and Ostermann, J{ö}rn},
booktitle = {Proceedings of the IEEE World Congress on Computational Intelligence},
keywords = {Curvature-Aware},
month = {07},
title = {Improved Compression of Artificial Neural Networks through Curvature-Aware Training},
year = 2022
}%0 Conference Paper
%1 HinLia2022
%A Hinrichs, Reemt
%A Liang, Kai
%A Lu, Ze
%A Ostermann, J{ö}rn
%B Proceedings of the IEEE World Congress on Computational Intelligence
%D 2022
%T Improved Compression of Artificial Neural Networks through Curvature-Aware Training - Benjamins, C., Jankovic, A., Raponi, E., van der Blom, K., Lindauer, M., and Doerr, C. (2022)Towards Automated Design of Bayesian Optimization via Exploratory Landscape Analysis. In Workshop on Meta-Learning (MetaLearn 2022).
@inproceedings{benjamins2022towards,
author = {Benjamins, Carolin and Jankovic, Anja and Raponi, Elena and van der Blom, Koen and Lindauer, Marius and Doerr, Carola},
booktitle = {Workshop on Meta-Learning (MetaLearn 2022)},
keywords = {leibnizailab},
title = {Towards Automated Design of Bayesian Optimization via Exploratory Landscape Analysis},
year = 2022
}%0 Conference Paper
%1 benjamins2022towards
%A Benjamins, Carolin
%A Jankovic, Anja
%A Raponi, Elena
%A van der Blom, Koen
%A Lindauer, Marius
%A Doerr, Carola
%B Workshop on Meta-Learning (MetaLearn 2022)
%D 2022
%T Towards Automated Design of Bayesian Optimization via Exploratory Landscape Analysis
%U https://www.ai.uni-hannover.de/de/forschung/publikationen/publikationen-detailansicht?tx_t3luhpublications_publications%5Baction%5D=show&tx_t3luhpublications_publications%5Bcontroller%5D=Publication&tx_t3luhpublications_publications%5Bpublication%5D=7783&cHash=04b8ffd50e56727ae4181c8e2a2261f1 - Chen, W.-F., Chen, M.-H., Mudgal, G., and Wachsmuth, H. (2022)Analyzing Culture-Specific Argument Structures in Learner Essays. In Proceedings of the 9th Workshop on Argument Mining (ArgMining 2022), pp. 51–61.
@inproceedings{Chen_Chen_Mudgal_Wachsmuth_2022,
author = {Chen, Wei-Fan and Chen, Mei-Hua and Mudgal, Garima and Wachsmuth, Henning},
booktitle = {Proceedings of the 9th Workshop on Argument Mining (ArgMining 2022)},
keywords = {leibnizailab},
pages = {51–61},
title = {Analyzing Culture-Specific Argument Structures in Learner Essays},
year = 2022
}%0 Conference Paper
%1 Chen_Chen_Mudgal_Wachsmuth_2022
%A Chen, Wei-Fan
%A Chen, Mei-Hua
%A Mudgal, Garima
%A Wachsmuth, Henning
%B Proceedings of the 9th Workshop on Argument Mining (ArgMining 2022)
%D 2022
%P 51–61
%T Analyzing Culture-Specific Argument Structures in Learner Essays - Biedenkapp, A., Speck, D., Sievers, S., Hutter, F., Lindauer, M., and Seipp, J. (2022)Learning Domain-Independent Policies for Open List Selection. In Proceedings of the 3rd ICAPS workshop on Bridging the Gap Between AI Planning and Reinforcement Learning (PRL), pp. 1–9.
@inproceedings{BieSpe2022,
author = {Biedenkapp, André and Speck, David and Sievers, Silvan and Hutter, Frank and Lindauer, Marius and Seipp, Jendrik},
booktitle = {Proceedings of the 3rd ICAPS workshop on Bridging the Gap Between AI Planning and Reinforcement Learning (PRL)},
keywords = {leibnizailab},
pages = {1-9},
title = {Learning Domain-Independent Policies for Open List Selection},
year = 2022
}%0 Conference Paper
%1 BieSpe2022
%A Biedenkapp, André
%A Speck, David
%A Sievers, Silvan
%A Hutter, Frank
%A Lindauer, Marius
%A Seipp, Jendrik
%B Proceedings of the 3rd ICAPS workshop on Bridging the Gap Between AI Planning and Reinforcement Learning (PRL)
%D 2022
%P 1-9
%T Learning Domain-Independent Policies for Open List Selection - Alshomary, M., El Baff, R., Gurcke, T., and Wachsmuth, H. (2022)The Moral Debater: A Study on the Computational Generation of Morally Framed Arguments. In Proceedings of the 60th Annual Meeting of the Association for Computational Linguistics, pp. 8782–8797.
@inproceedings{alshomary2022moral,
author = {Alshomary, Milad and El Baff, Roxanne and Gurcke, Timon and Wachsmuth, Henning},
booktitle = {Proceedings of the 60th Annual Meeting of the Association for Computational Linguistics},
keywords = {leibnizailab},
pages = {8782–8797},
title = {The Moral Debater: A Study on the Computational Generation of Morally Framed Arguments},
year = 2022
}%0 Conference Paper
%1 alshomary2022moral
%A Alshomary, Milad
%A El Baff, Roxanne
%A Gurcke, Timon
%A Wachsmuth, Henning
%B Proceedings of the 60th Annual Meeting of the Association for Computational Linguistics
%D 2022
%P 8782–8797
%T The Moral Debater: A Study on the Computational Generation of Morally Framed Arguments - Parker-Holder, J., Rajan, R., Song, X., Biedenkapp, A., Miao, Y., Eimer, T., Zhang, B., Nguyen, V., Calandra, R., Faust, A., Hutter, F., and Lindauer, M. (2022)Automated Reinforcement Learning (AutoRL): A Survey and Open Problems, Journal of Artificial Intelligence Research 74 (2022) 517–568.
@article{2201.03916,
author = {Parker-Holder, Jack and Rajan, Raghu and Song, Xingyou and Biedenkapp, André and Miao, Yingjie and Eimer, Theresa and Zhang, Baohe and Nguyen, Vu and Calandra, Roberto and Faust, Aleksandra and Hutter, Frank and Lindauer, Marius},
journal = {Journal of Artificial Intelligence Research 74 (2022)},
keywords = {AutoML},
pages = {517-568},
title = {Automated Reinforcement Learning (AutoRL): A Survey and Open Problems},
year = 2022
}%0 Journal Article
%1 2201.03916
%A Parker-Holder, Jack
%A Rajan, Raghu
%A Song, Xingyou
%A Biedenkapp, André
%A Miao, Yingjie
%A Eimer, Theresa
%A Zhang, Baohe
%A Nguyen, Vu
%A Calandra, Roberto
%A Faust, Aleksandra
%A Hutter, Frank
%A Lindauer, Marius
%D 2022
%J Journal of Artificial Intelligence Research 74 (2022)
%P 517-568
%R 10.1613/jair.1.13596
%T Automated Reinforcement Learning (AutoRL): A Survey and Open Problems - Benjamins, C., Eimer, T., Schubert, F., Mohan, A., Biedenkapp, A., Rosenhahn, B., Hutter, F., and Lindauer, M. (2022)Contextualize Me -- The Case for Context in Reinforcement Learning, Arxiv Preprint, arXiv.
@article{https://doi.org/10.48550/arxiv.2202.04500,
author = {Benjamins, Carolin and Eimer, Theresa and Schubert, Frederik and Mohan, Aditya and Biedenkapp, André and Rosenhahn, Bodo and Hutter, Frank and Lindauer, Marius},
journal = {Arxiv Preprint},
keywords = {leibnizailab},
publisher = {arXiv},
title = {Contextualize Me -- The Case for Context in Reinforcement Learning},
year = 2022
}%0 Journal Article
%1 https://doi.org/10.48550/arxiv.2202.04500
%A Benjamins, Carolin
%A Eimer, Theresa
%A Schubert, Frederik
%A Mohan, Aditya
%A Biedenkapp, André
%A Rosenhahn, Bodo
%A Hutter, Frank
%A Lindauer, Marius
%D 2022
%I arXiv
%J Arxiv Preprint
%R 10.48550/ARXIV.2202.04500
%T Contextualize Me -- The Case for Context in Reinforcement Learning
%U https://arxiv.org/abs/2202.04500 - Reinders, C., Schubert, F., and Rosenhahn, B. (2022)ChimeraMix: Image Classification on Small Datasets via Masked Feature Mixing. In Arxiv Preprint.
@inproceedings{ReiSch2022,
author = {Reinders, Christoph and Schubert, Frederik and Rosenhahn, Bodo},
booktitle = {Arxiv Preprint},
keywords = {Feature},
month = {03},
title = {ChimeraMix: Image Classification on Small Datasets via Masked Feature Mixing},
year = 2022
}%0 Conference Paper
%1 ReiSch2022
%A Reinders, Christoph
%A Schubert, Frederik
%A Rosenhahn, Bodo
%B Arxiv Preprint
%D 2022
%T ChimeraMix: Image Classification on Small Datasets via Masked Feature Mixing - Grimm, E., Kuhnke, F., Gajdt, A., Ostermann, J., and Knoche, M. (2022)Accurate Quantification of Anthocyanin in Red Flesh Apples Using Digital Photography and Image Analysis, Horticulturae 8.
@article{GriKuh2022,
author = {Grimm, Eckhard and Kuhnke, Felix and Gajdt, Anna and Ostermann, J{ö}rn and Knoche, Moritz},
journal = {Horticulturae},
keywords = {Flesh},
month = {01},
number = 2,
title = {Accurate Quantification of Anthocyanin in Red Flesh Apples Using Digital Photography and Image Analysis},
volume = 8,
year = 2022
}%0 Journal Article
%1 GriKuh2022
%A Grimm, Eckhard
%A Kuhnke, Felix
%A Gajdt, Anna
%A Ostermann, J{ö}rn
%A Knoche, Moritz
%D 2022
%J Horticulturae
%N 2
%R https://doi.org/10.3390/horticulturae8020145
%T Accurate Quantification of Anthocyanin in Red Flesh Apples Using Digital Photography and Image Analysis
%U https://www.mdpi.com/2311-7524/8/2/145
%V 8 - Zhao, B., van der Aa, H., Nguyen, T. T., Nguyen, Q. V. H., and Weidlich, M. (2021){EIRES}: Efficient Integration of Remote Data in Event Stream Processing. In Proceedings of the 2021 International Conference on Management of Data, {ACM}.To support reactive and predictive applications, complex event processing (CEP) systems detect patterns in event streams based on predefined queries. To determine the events that constitute a query match, their payload data may need to be assessed together with data from remote sources. Such dependencies are problematic, since waiting for remote data to be fetched interrupts the processing of the stream. Yet, without event selection based on remote data, the query state to maintain may grow exponentially. In either case, the performance of the CEP system degrades drastically. To tackle these issues, we present EIRES, a framework for efficient integration of static data from remote sources in CEP. It employs a cost-model to determine when to fetch certain remote data elements and how long to keep them in a cache for future use. EIRES combines strategies for (i) prefetching that queries remote data based on anticipated use and (ii) lazy evaluation that postpones the event selection based on remote data without interrupting the stream processing. Our experiments indicate that the combination of these strategies improves the latency of query evaluation by up to 3,725x for synthetic data and 47x for real-world data.
@inproceedings{Zhao_2021,
abstract = {To support reactive and predictive applications, complex event processing (CEP) systems detect patterns in event streams based on predefined queries. To determine the events that constitute a query match, their payload data may need to be assessed together with data from remote sources. Such dependencies are problematic, since waiting for remote data to be fetched interrupts the processing of the stream. Yet, without event selection based on remote data, the query state to maintain may grow exponentially. In either case, the performance of the CEP system degrades drastically. To tackle these issues, we present EIRES, a framework for efficient integration of static data from remote sources in CEP. It employs a cost-model to determine when to fetch certain remote data elements and how long to keep them in a cache for future use. EIRES combines strategies for (i) prefetching that queries remote data based on anticipated use and (ii) lazy evaluation that postpones the event selection based on remote data without interrupting the stream processing. Our experiments indicate that the combination of these strategies improves the latency of query evaluation by up to 3,725x for synthetic data and 47x for real-world data.},
author = {Zhao, Bo and van der Aa, Han and Nguyen, Thanh Tam and Nguyen, Quoc Viet Hung and Weidlich, Matthias},
booktitle = {Proceedings of the 2021 International Conference on Management of Data},
keywords = {l3s},
month = {06},
publisher = {{ACM}},
title = {{EIRES}: Efficient Integration of Remote Data in Event Stream Processing},
year = 2021
}%0 Conference Paper
%1 Zhao_2021
%A Zhao, Bo
%A van der Aa, Han
%A Nguyen, Thanh Tam
%A Nguyen, Quoc Viet Hung
%A Weidlich, Matthias
%B Proceedings of the 2021 International Conference on Management of Data
%D 2021
%I {ACM}
%R 10.1145/3448016.3457304
%T {EIRES}: Efficient Integration of Remote Data in Event Stream Processing
%U https://doi.org/10.1145%2F3448016.3457304
%X To support reactive and predictive applications, complex event processing (CEP) systems detect patterns in event streams based on predefined queries. To determine the events that constitute a query match, their payload data may need to be assessed together with data from remote sources. Such dependencies are problematic, since waiting for remote data to be fetched interrupts the processing of the stream. Yet, without event selection based on remote data, the query state to maintain may grow exponentially. In either case, the performance of the CEP system degrades drastically. To tackle these issues, we present EIRES, a framework for efficient integration of static data from remote sources in CEP. It employs a cost-model to determine when to fetch certain remote data elements and how long to keep them in a cache for future use. EIRES combines strategies for (i) prefetching that queries remote data based on anticipated use and (ii) lazy evaluation that postpones the event selection based on remote data without interrupting the stream processing. Our experiments indicate that the combination of these strategies improves the latency of query evaluation by up to 3,725x for synthetic data and 47x for real-world data. - Dockhorn, A., and Kruse, R. (2021)Modelheuristics for efficient forward model learning, At-Automatisierungstechnik.
@article{DocKru2021a,
author = {Dockhorn, Alexander and Kruse, Rudolf},
journal = {At-Automatisierungstechnik},
keywords = {for},
month = 10,
title = {Modelheuristics for efficient forward model learning},
year = 2021
}%0 Journal Article
%1 DocKru2021a
%A Dockhorn, Alexander
%A Kruse, Rudolf
%D 2021
%J At-Automatisierungstechnik
%R 10.1515/auto-2021-0037
%T Modelheuristics for efficient forward model learning
%U https://www.degruyter.com/document/doi/10.1515/auto-2021-0037/html - Kaushal, A., Saha, A., and Ganguly, N. (2021)tWT–WT: A Dataset to Assert the Role of Target Entities for Detecting Stance of Tweets, pp. 3879–3889.The stance detection task aims at detecting the stance of a tweet or a text for a target. These targets can be named entities or free-form sentences (claims). Though the task involves reasoning of the tweet with respect to a target, we find that it is possible to achieve high accuracy on several publicly available Twitter stance detection datasets without looking at the target sentence. Specifically, a simple tweet classification model achieved human-level performance on the WT–WT dataset and more than two-third accuracy on various other datasets. We investigate the existence of biases in such datasets to find the potential spurious correlations of sentiment-stance relations and lexical choice associated with the stance category. Furthermore, we propose a new large dataset free of such biases and demonstrate its aptness on the existing stance detection systems. Our empirical findings show much scope for research on the stance detection task and proposes several considerations for creating future stance detection datasets.
@proceedings{J,
abstract = {The stance detection task aims at detecting the stance of a tweet or a text for a target. These targets can be named entities or free-form sentences (claims). Though the task involves reasoning of the tweet with respect to a target, we find that it is possible to achieve high accuracy on several publicly available Twitter stance detection datasets without looking at the target sentence. Specifically, a simple tweet classification model achieved human-level performance on the WT–WT dataset and more than two-third accuracy on various other datasets. We investigate the existence of biases in such datasets to find the potential spurious correlations of sentiment-stance relations and lexical choice associated with the stance category. Furthermore, we propose a new large dataset free of such biases and demonstrate its aptness on the existing stance detection systems. Our empirical findings show much scope for research on the stance detection task and proposes several considerations for creating future stance detection datasets.},
author = {Kaushal, Ayush and Saha, Avirup and Ganguly, Niloy},
keywords = {leibnizailab},
month = {06},
pages = {3879-3889},
title = {tWT–WT: A Dataset to Assert the Role of Target Entities for Detecting Stance of Tweets},
year = 2021
}%0 Conference Proceedings
%1 J
%A Kaushal, Ayush
%A Saha, Avirup
%A Ganguly, Niloy
%D 2021
%P 3879-3889
%T tWT–WT: A Dataset to Assert the Role of Target Entities for Detecting Stance of Tweets
%X The stance detection task aims at detecting the stance of a tweet or a text for a target. These targets can be named entities or free-form sentences (claims). Though the task involves reasoning of the tweet with respect to a target, we find that it is possible to achieve high accuracy on several publicly available Twitter stance detection datasets without looking at the target sentence. Specifically, a simple tweet classification model achieved human-level performance on the WT–WT dataset and more than two-third accuracy on various other datasets. We investigate the existence of biases in such datasets to find the potential spurious correlations of sentiment-stance relations and lexical choice associated with the stance category. Furthermore, we propose a new large dataset free of such biases and demonstrate its aptness on the existing stance detection systems. Our empirical findings show much scope for research on the stance detection task and proposes several considerations for creating future stance detection datasets. - Pestel-Schiller, U., Hu, K., Gritzner, D., and Ostermann, J. (2021)Determination of Relevant Hyperspectral Bands Using a Spectrally Constrained CNN. In 11th Workshop on Hyperspectral Imaging and Signal Processing: Evolution in Remote Sensing (WHISPERS), Paper 15.
@inproceedings{PesHu2021a,
author = {Pestel-Schiller, Ulrike and Hu, Kai and Gritzner, Daniel and Ostermann, J{ö}rn},
booktitle = {11th Workshop on Hyperspectral Imaging and Signal Processing: Evolution in Remote Sensing (WHISPERS), Paper 15},
keywords = {Relevant},
month = {03},
title = {Determination of Relevant Hyperspectral Bands Using a Spectrally Constrained CNN},
year = 2021
}%0 Conference Paper
%1 PesHu2021a
%A Pestel-Schiller, Ulrike
%A Hu, Kai
%A Gritzner, Daniel
%A Ostermann, J{ö}rn
%B 11th Workshop on Hyperspectral Imaging and Signal Processing: Evolution in Remote Sensing (WHISPERS), Paper 15
%D 2021
%T Determination of Relevant Hyperspectral Bands Using a Spectrally Constrained CNN - Rumberg, L., Ehlert, H., L{ü}dtke, U., and Ostermann, J. (2021)Age-Invariant Training for End-to-End Child Speech Recognition using Adversarial Multi-Task Learning. In Proceedings INTERSPEECH 2021 -- 22th Annual Conference of the International Speech Communication Association.
@inproceedings{RumEhl2021,
author = {Rumberg, Lars and Ehlert, Hanna and L{ü}dtke, Ulrike and Ostermann, J{ö}rn},
booktitle = {Proceedings INTERSPEECH 2021 -- 22th Annual Conference of the International Speech Communication Association},
keywords = {Recognition},
month = {08},
title = {Age-Invariant Training for End-to-End Child Speech Recognition using Adversarial Multi-Task Learning},
year = 2021
}%0 Conference Paper
%1 RumEhl2021
%A Rumberg, Lars
%A Ehlert, Hanna
%A L{ü}dtke, Ulrike
%A Ostermann, J{ö}rn
%B Proceedings INTERSPEECH 2021 -- 22th Annual Conference of the International Speech Communication Association
%D 2021
%T Age-Invariant Training for End-to-End Child Speech Recognition using Adversarial Multi-Task Learning - Dockhorn, A., Hurtado-Grueso, J., Jeurissen, D., Xu, L., and Perez-Liebana, D. (2021)Portfolio Search and Optimization for General Strategy Game-Playing. In 2021 IEEE Congress on Evolutionary Computation (CEC), pp. 2085–2092.
@inproceedings{DocHur2021,
author = {Dockhorn, Alexander and Hurtado-Grueso, Jorge and Jeurissen, Dominik and Xu, Linjie and Perez-Liebana, Diego},
booktitle = {2021 IEEE Congress on Evolutionary Computation (CEC)},
keywords = {Search},
pages = {2085-2092},
title = {Portfolio Search and Optimization for General Strategy Game-Playing},
year = 2021
}%0 Conference Paper
%1 DocHur2021
%A Dockhorn, Alexander
%A Hurtado-Grueso, Jorge
%A Jeurissen, Dominik
%A Xu, Linjie
%A Perez-Liebana, Diego
%B 2021 IEEE Congress on Evolutionary Computation (CEC)
%D 2021
%P 2085-2092
%R 10.1109/CEC45853.2021.9504824
%T Portfolio Search and Optimization for General Strategy Game-Playing
%U https://ieeexplore.ieee.org/document/9504824 - Eimer, T., Biedenkapp, A., Reimer, M., Adriaensen, S., Hutter, F., and Lindauer, M. (2021)DACBench: A Benchmark Library for Dynamic Algorithm Configuration. In Proceedings of the international joint conference on artificial intelligence (IJCAI).
@inproceedings{EimBie2021b,
author = {Eimer, Theresa and Biedenkapp, André and Reimer, Maximilian and Adriaensen, Steven and Hutter, Frank and Lindauer, Marius},
booktitle = {Proceedings of the international joint conference on artificial intelligence (IJCAI)},
keywords = {leibnizailab},
month = {08},
note = {To appear.},
title = {DACBench: A Benchmark Library for Dynamic Algorithm Configuration},
year = 2021
}%0 Conference Paper
%1 EimBie2021b
%A Eimer, Theresa
%A Biedenkapp, André
%A Reimer, Maximilian
%A Adriaensen, Steven
%A Hutter, Frank
%A Lindauer, Marius
%B Proceedings of the international joint conference on artificial intelligence (IJCAI)
%D 2021
%T DACBench: A Benchmark Library for Dynamic Algorithm Configuration
%U https://arxiv.org/abs/2105.08541 - Hachmann, H., Kr{ü}ger, B., Rosenhahn, B., and Nogueira, W. (2021)Localization of Cochlear Implant Electrodes from Cone Beam Computed Tomography using Particle Belief Propagation. In International Symposium on Biomedical Imaging, ISBI.
@inproceedings{HacKru2021a,
author = {Hachmann, Hendrik and Kr{ü}ger, Benjamin and Rosenhahn, Bodo and Nogueira, Waldo},
booktitle = {International Symposium on Biomedical Imaging, ISBI},
keywords = {Computed},
month = {04},
title = {Localization of Cochlear Implant Electrodes from Cone Beam Computed Tomography using Particle Belief Propagation},
year = 2021
}%0 Conference Paper
%1 HacKru2021a
%A Hachmann, Hendrik
%A Kr{ü}ger, Benjamin
%A Rosenhahn, Bodo
%A Nogueira, Waldo
%B International Symposium on Biomedical Imaging, ISBI
%D 2021
%T Localization of Cochlear Implant Electrodes from Cone Beam Computed Tomography using Particle Belief Propagation
%U https://arxiv.org/abs/2103.10434 - Guerrero-Viu, J., Hauns, S., Izquierdo, S., Miotto, G., Schrodi, S., Biedenkapp, A., Elsken, T., Deng, D., Lindauer, M., and Hutter, F. (2021)Bag of Baselines for Multi-objective Joint Neural Architecture Search and Hyperparameter Optimization. In Proceedings of the international workshop on Automated Machine Learning (AutoML) at ICML’21.
@inproceedings{GueHau2021a,
author = {Guerrero-Viu, Julia and Hauns, Sven and Izquierdo, Sergio and Miotto, Guilherme and Schrodi, Simon and Biedenkapp, Andre and Elsken, Thomas and Deng, Difan and Lindauer, Marius and Hutter, Frank},
booktitle = {Proceedings of the international workshop on Automated Machine Learning (AutoML) at ICML'21},
keywords = {Optimization},
month = {07},
title = {Bag of Baselines for Multi-objective Joint Neural Architecture Search and Hyperparameter Optimization},
year = 2021
}%0 Conference Paper
%1 GueHau2021a
%A Guerrero-Viu, Julia
%A Hauns, Sven
%A Izquierdo, Sergio
%A Miotto, Guilherme
%A Schrodi, Simon
%A Biedenkapp, Andre
%A Elsken, Thomas
%A Deng, Difan
%A Lindauer, Marius
%A Hutter, Frank
%B Proceedings of the international workshop on Automated Machine Learning (AutoML) at ICML'21
%D 2021
%T Bag of Baselines for Multi-objective Joint Neural Architecture Search and Hyperparameter Optimization
%U https://arxiv.org/abs/2105.01015 - Schubert, F., Eimer, T., Rosenhahn, B., and Lindauer, M. (2021)Towards Automatic Risk Adaption in Distributional Reinforcement Learning. In Reinforcement Learning for Real Life (RL4RealLife) Workshop in the 38th International Conference on Machine Learning (ICML).
@inproceedings{SchEim2021b,
author = {Schubert, Frederik and Eimer, Theresa and Rosenhahn, Bodo and Lindauer, Marius},
booktitle = {Reinforcement Learning for Real Life (RL4RealLife) Workshop in the 38th International Conference on Machine Learning (ICML)},
keywords = {Reinforcement},
month = {07},
title = {Towards Automatic Risk Adaption in Distributional Reinforcement Learning},
year = 2021
}%0 Conference Paper
%1 SchEim2021b
%A Schubert, Frederik
%A Eimer, Theresa
%A Rosenhahn, Bodo
%A Lindauer, Marius
%B Reinforcement Learning for Real Life (RL4RealLife) Workshop in the 38th International Conference on Machine Learning (ICML)
%D 2021
%T Towards Automatic Risk Adaption in Distributional Reinforcement Learning
%U https://arxiv.org/abs/2106.06317 - Eimer, T., Biedenkapp, A., Hutter, F., and Lindauer, M. (2021)Self-Paced Context Evaluation for Contextual Reinforcement Learning. In Proceedings of the international conference on machine learning (ICML).
@inproceedings{EimBie2021a,
author = {Eimer, Theresa and Biedenkapp, Andre and Hutter, Frank and Lindauer, Marius},
booktitle = {Proceedings of the international conference on machine learning (ICML)},
keywords = {Reinforcement},
month = {07},
note = {To appear},
title = {Self-Paced Context Evaluation for Contextual Reinforcement Learning},
year = 2021
}%0 Conference Paper
%1 EimBie2021a
%A Eimer, Theresa
%A Biedenkapp, Andre
%A Hutter, Frank
%A Lindauer, Marius
%B Proceedings of the international conference on machine learning (ICML)
%D 2021
%T Self-Paced Context Evaluation for Contextual Reinforcement Learning
%U https://arxiv.org/abs/2106.05110 - Narisetti, N., Henke, M., Seiler, C., Junker, A., Ostermann, J., Altmann, T., and Gladilin, E. (2021)Fully-automated root image analysis (faRIA), Scientific Reports 11.
@article{NarHen2021,
author = {Narisetti, Narendra and Henke, Michael and Seiler, Christiane and Junker, Astrid and Ostermann, J{ö}rn and Altmann, Thomas and Gladilin, Evgeny},
journal = {Scientific Reports},
keywords = {analysis},
month = {08},
title = {Fully-automated root image analysis (faRIA)},
volume = 11,
year = 2021
}%0 Journal Article
%1 NarHen2021
%A Narisetti, Narendra
%A Henke, Michael
%A Seiler, Christiane
%A Junker, Astrid
%A Ostermann, J{ö}rn
%A Altmann, Thomas
%A Gladilin, Evgeny
%D 2021
%J Scientific Reports
%R https://doi.org/10.1038/s41598-021-95480-y
%T Fully-automated root image analysis (faRIA)
%U https://www.nature.com/articles/s41598-021-95480-y
%V 11 - Wu, W., Li, B., Luo, C., and Nejdl, W. (2021)Hashing-Accelerated Graph Neural Networks for Link Prediction. In .Networks are ubiquitous in the real world. Link prediction, as one of the key problems for network-structured data, aims to predict whether there exists a link between two nodes. The traditional approaches are based on the explicit similarity computation between the compact node representation by embedding each node into a low-dimensional space. In order to efficiently handle the intensive similarity computation in link prediction, the hashing technique has been successfully used to produce the node representation in the Hamming space. However, the hashing-based link prediction algorithms face accuracy loss from the randomized hashing techniques or inefficiency from the learning to hash techniques in the embedding process. Currently, the Graph Neural Network (GNN) framework has been widely applied to the graph-related tasks in an end-to-end manner, but it commonly requires substantial computational resources and memory costs due to massive parameter learning, which makes the GNN-based algorithms impractical without the help of a powerful workhorse. In this paper, we propose a simple and effective model called #GNN, which balances the trade-off between accuracy and efficiency. #GNN is able to efficiently acquire node representation in the Hamming space for link prediction by exploiting the randomized hashing technique to implement message passing and capture high-order proximity in the GNN framework. Furthermore, we characterize the discriminative power of #GNN in probability. The extensive experimental results demonstrate that the proposed #GNN algorithm achieves accuracy comparable to the learning-based algorithms and outperforms the randomized algorithm, while running significantly faster than the learning-based algorithms. Also, the proposed algorithm shows excellent scalability on a large-scale network with the limited resources.
@conference{wu2021hashingaccelerated,
abstract = {Networks are ubiquitous in the real world. Link prediction, as one of the key problems for network-structured data, aims to predict whether there exists a link between two nodes. The traditional approaches are based on the explicit similarity computation between the compact node representation by embedding each node into a low-dimensional space. In order to efficiently handle the intensive similarity computation in link prediction, the hashing technique has been successfully used to produce the node representation in the Hamming space. However, the hashing-based link prediction algorithms face accuracy loss from the randomized hashing techniques or inefficiency from the learning to hash techniques in the embedding process. Currently, the Graph Neural Network (GNN) framework has been widely applied to the graph-related tasks in an end-to-end manner, but it commonly requires substantial computational resources and memory costs due to massive parameter learning, which makes the GNN-based algorithms impractical without the help of a powerful workhorse. In this paper, we propose a simple and effective model called #GNN, which balances the trade-off between accuracy and efficiency. #GNN is able to efficiently acquire node representation in the Hamming space for link prediction by exploiting the randomized hashing technique to implement message passing and capture high-order proximity in the GNN framework. Furthermore, we characterize the discriminative power of #GNN in probability. The extensive experimental results demonstrate that the proposed #GNN algorithm achieves accuracy comparable to the learning-based algorithms and outperforms the randomized algorithm, while running significantly faster than the learning-based algorithms. Also, the proposed algorithm shows excellent scalability on a large-scale network with the limited resources.},
author = {Wu, Wei and Li, Bin and Luo, Chuan and Nejdl, Wolfgang},
keywords = {l3s},
note = {cite arxiv:2105.14280},
title = {Hashing-Accelerated Graph Neural Networks for Link Prediction},
year = 2021
}%0 Generic
%1 wu2021hashingaccelerated
%A Wu, Wei
%A Li, Bin
%A Luo, Chuan
%A Nejdl, Wolfgang
%D 2021
%R 10.1145/3442381.3449884
%T Hashing-Accelerated Graph Neural Networks for Link Prediction
%U http://arxiv.org/abs/2105.14280
%X Networks are ubiquitous in the real world. Link prediction, as one of the key problems for network-structured data, aims to predict whether there exists a link between two nodes. The traditional approaches are based on the explicit similarity computation between the compact node representation by embedding each node into a low-dimensional space. In order to efficiently handle the intensive similarity computation in link prediction, the hashing technique has been successfully used to produce the node representation in the Hamming space. However, the hashing-based link prediction algorithms face accuracy loss from the randomized hashing techniques or inefficiency from the learning to hash techniques in the embedding process. Currently, the Graph Neural Network (GNN) framework has been widely applied to the graph-related tasks in an end-to-end manner, but it commonly requires substantial computational resources and memory costs due to massive parameter learning, which makes the GNN-based algorithms impractical without the help of a powerful workhorse. In this paper, we propose a simple and effective model called #GNN, which balances the trade-off between accuracy and efficiency. #GNN is able to efficiently acquire node representation in the Hamming space for link prediction by exploiting the randomized hashing technique to implement message passing and capture high-order proximity in the GNN framework. Furthermore, we characterize the discriminative power of #GNN in probability. The extensive experimental results demonstrate that the proposed #GNN algorithm achieves accuracy comparable to the learning-based algorithms and outperforms the randomized algorithm, while running significantly faster than the learning-based algorithms. Also, the proposed algorithm shows excellent scalability on a large-scale network with the limited resources. - Holzapfel, C., Sag, S., Graf-Schindler, J., Fischer, M., Drabsch, T., Illig, T., Grallert, H., Stecher, L., Strack, C., Caterson, I., Jebb, S., Hauner, H., and Baessler, A. (2021)Association between single nucleotide polymorphisms and weight reduction in behavioural interventions—a pooled analysis, Nutrients, MDPI 13.Knowledge of the association between single nucleotide polymorphisms (SNPs) and weight loss is limited. The aim was to analyse whether selected obesity-associated SNPs within the fat mass and obesity-associated (FTO), transmembrane protein 18 (TMEM18), melanocortin-4 receptor (MC4R), SEC16 homolog B (SEC16B), and brain-derived neurotrophic factor (BDNF) gene are associated with anthropometric changes during behavioural intervention for weight loss. genetic and anthropometric data from 576 individuals with overweight and obesity from four lifestyle interventions were obtained. A genetic predisposition score (GPS) was calculated. Our results show that study participants had a mean age of 48.2 ± 12.6 years and a mean baseline body mass index of 33.9 ± 6.4 kg/m2. Mean weight reduction after 12 months was −7.7 ± 10.9 kg. After 12 months of intervention, the MC4R SNPs rs571312 and rs17782313 were significantly associated with a greater decrease in body weight and BMI (p = 0.012, p = 0.011, respectively). The investigated SNPs within the other four genetic loci showed no statistically significant association with changes in anthropometric parameters. The GPS showed no statistically significant association with weight reduction. In conclusion there was no consistent evidence for statistically significant associations of SNPs with anthropometric changes during a behavioural intervention. It seems that other factors play a more significant in weight management than the investigated SNPs.
@article{holzapfel2021association,
abstract = {Knowledge of the association between single nucleotide polymorphisms (SNPs) and weight loss is limited. The aim was to analyse whether selected obesity-associated SNPs within the fat mass and obesity-associated (FTO), transmembrane protein 18 (TMEM18), melanocortin-4 receptor (MC4R), SEC16 homolog B (SEC16B), and brain-derived neurotrophic factor (BDNF) gene are associated with anthropometric changes during behavioural intervention for weight loss. genetic and anthropometric data from 576 individuals with overweight and obesity from four lifestyle interventions were obtained. A genetic predisposition score (GPS) was calculated. Our results show that study participants had a mean age of 48.2 ± 12.6 years and a mean baseline body mass index of 33.9 ± 6.4 kg/m2. Mean weight reduction after 12 months was −7.7 ± 10.9 kg. After 12 months of intervention, the MC4R SNPs rs571312 and rs17782313 were significantly associated with a greater decrease in body weight and BMI (p = 0.012, p = 0.011, respectively). The investigated SNPs within the other four genetic loci showed no statistically significant association with changes in anthropometric parameters. The GPS showed no statistically significant association with weight reduction. In conclusion there was no consistent evidence for statistically significant associations of SNPs with anthropometric changes during a behavioural intervention. It seems that other factors play a more significant in weight management than the investigated SNPs.},
author = {Holzapfel, C and Sag, S and Graf-Schindler, J and Fischer, M and Drabsch, T and Illig, T and Grallert, H and Stecher, L and Strack, C and Caterson, ID and Jebb, SA and Hauner, H and Baessler, A},
journal = {Nutrients},
keywords = {l3s},
number = 3,
publisher = {MDPI},
title = {Association between single nucleotide polymorphisms and weight reduction in behavioural interventions—a pooled analysis},
type = {Publication},
volume = 13,
year = 2021
}%0 Journal Article
%1 holzapfel2021association
%A Holzapfel, C
%A Sag, S
%A Graf-Schindler, J
%A Fischer, M
%A Drabsch, T
%A Illig, T
%A Grallert, H
%A Stecher, L
%A Strack, C
%A Caterson, ID
%A Jebb, SA
%A Hauner, H
%A Baessler, A
%D 2021
%I MDPI
%J Nutrients
%N 3
%R 10.3390/nu13030819
%T Association between single nucleotide polymorphisms and weight reduction in behavioural interventions—a pooled analysis
%U https://ora.ox.ac.uk/objects/uuid:98987391-ba79-4686-a819-5fbe2a79ff45
%V 13
%X Knowledge of the association between single nucleotide polymorphisms (SNPs) and weight loss is limited. The aim was to analyse whether selected obesity-associated SNPs within the fat mass and obesity-associated (FTO), transmembrane protein 18 (TMEM18), melanocortin-4 receptor (MC4R), SEC16 homolog B (SEC16B), and brain-derived neurotrophic factor (BDNF) gene are associated with anthropometric changes during behavioural intervention for weight loss. genetic and anthropometric data from 576 individuals with overweight and obesity from four lifestyle interventions were obtained. A genetic predisposition score (GPS) was calculated. Our results show that study participants had a mean age of 48.2 ± 12.6 years and a mean baseline body mass index of 33.9 ± 6.4 kg/m2. Mean weight reduction after 12 months was −7.7 ± 10.9 kg. After 12 months of intervention, the MC4R SNPs rs571312 and rs17782313 were significantly associated with a greater decrease in body weight and BMI (p = 0.012, p = 0.011, respectively). The investigated SNPs within the other four genetic loci showed no statistically significant association with changes in anthropometric parameters. The GPS showed no statistically significant association with weight reduction. In conclusion there was no consistent evidence for statistically significant associations of SNPs with anthropometric changes during a behavioural intervention. It seems that other factors play a more significant in weight management than the investigated SNPs. - Dockhorn, A., Grueso, J. H., Jeurissen, D., and Perez-Liebana, D. (2020)“Stratega”: A General Strategy Games Framework. In Joint Proceedings of the AIIDE 2020 Workshops co-located with 16th AAAI Conference on Artificial Intelligence and Interactive Digital Entertainment (AIIDE 2020); Artificial Intelligence for Strategy Games, pp. 1–7.
@inproceedings{DocGru2020,
author = {Dockhorn, Alexander and Grueso, Jorge Hurtado and Jeurissen, Dominik and Perez-Liebana, Diego},
booktitle = {Joint Proceedings of the AIIDE 2020 Workshops co-located with 16th AAAI Conference on Artificial Intelligence and Interactive Digital Entertainment (AIIDE 2020); Artificial Intelligence for Strategy Games},
keywords = {“Stratega”},
pages = {1--7},
title = {“Stratega”: A General Strategy Games Framework},
year = 2020
}%0 Conference Paper
%1 DocGru2020
%A Dockhorn, Alexander
%A Grueso, Jorge Hurtado
%A Jeurissen, Dominik
%A Perez-Liebana, Diego
%B Joint Proceedings of the AIIDE 2020 Workshops co-located with 16th AAAI Conference on Artificial Intelligence and Interactive Digital Entertainment (AIIDE 2020); Artificial Intelligence for Strategy Games
%D 2020
%P 1--7
%T “Stratega”: A General Strategy Games Framework
%U http://ceur-ws.org/Vol-2862/ - Dockhorn, A. (2020)Vorhersagebasierte Suche f{ü}r autonomes Spielen, pp. 69–78, GI.
@book{Doc2020,
author = {Dockhorn, Alexander},
keywords = {Vorhersagebasierte},
pages = {69-78},
publisher = {GI},
title = {Vorhersagebasierte Suche f{ü}r autonomes Spielen},
year = 2020
}%0 Book
%1 Doc2020
%A Dockhorn, Alexander
%D 2020
%I GI
%P 69-78
%R 20.500.12116/37928
%T Vorhersagebasierte Suche f{ü}r autonomes Spielen
%U https://dl.gi.de/20.500.12116/37928
%@ 978-3-88579-775-3 - Dockhorn, A., and Kruse, R. (2020)Forward Model Learning for Motion Control Tasks. In 2020 IEEE 10th International Conference on Intelligent Systems (IS), pp. 1–5.
@inproceedings{DocKru2020b,
author = {Dockhorn, Alexander and Kruse, Rudolf},
booktitle = {2020 IEEE 10th International Conference on Intelligent Systems (IS)},
keywords = {Model},
month = {09},
pages = {1--5},
title = {Forward Model Learning for Motion Control Tasks},
year = 2020
}%0 Conference Paper
%1 DocKru2020b
%A Dockhorn, Alexander
%A Kruse, Rudolf
%B 2020 IEEE 10th International Conference on Intelligent Systems (IS)
%D 2020
%P 1--5
%R 10.1109/IS48319.2020.9199978
%T Forward Model Learning for Motion Control Tasks
%U https://ieeexplore.ieee.org/document/9199978
%@ 9781728154565 - Hu, T., Iosifidis, V., Liao, W., Zhang, H., Yang, M. Y., Ntoutsi, E., and Rosenhahn, B. (2020)FairNN - Conjoint Learning of Fair Representations for Fair Decisions.. In Discovery Science, pp. 581–595, Springer International Publishing.
@incollection{Hu_2020,
author = {Hu, Tongxin and Iosifidis, Vasileios and Liao, Wentong and Zhang, Hang and Yang, Michael Ying and Ntoutsi, Eirini and Rosenhahn, Bodo},
booktitle = {Discovery Science},
keywords = {l3s},
pages = {581-595},
publisher = {Springer International Publishing},
series = {Lecture Notes in Computer Science},
title = {FairNN - Conjoint Learning of Fair Representations for Fair Decisions.},
type = {Publication},
volume = 12323,
year = 2020
}%0 Book Section
%1 Hu_2020
%A Hu, Tongxin
%A Iosifidis, Vasileios
%A Liao, Wentong
%A Zhang, Hang
%A Yang, Michael Ying
%A Ntoutsi, Eirini
%A Rosenhahn, Bodo
%B Discovery Science
%D 2020
%I Springer International Publishing
%P 581-595
%R 10.1007/978-3-030-61527-7_38
%T FairNN - Conjoint Learning of Fair Representations for Fair Decisions.
%U https://doi.org/10.1007%2F978-3-030-61527-7_38
%V 12323 - Dockhorn, A., Schwensfeier, T., and Kruse, R. (2019)Fuzzy Multiset Clustering for Metagame Analysis. In Proceedings of the 11th Conference of the European Society for Fuzzy Logic and Technology (EUSFLAT 2019), pp. 536–543.
@inproceedings{DocSch2019a,
author = {Dockhorn, Alexander and Schwensfeier, Tony and Kruse, Rudolf},
booktitle = {Proceedings of the 11th Conference of the European Society for Fuzzy Logic and Technology (EUSFLAT 2019)},
keywords = {Multiset},
month = {08},
pages = {536-543},
title = {Fuzzy Multiset Clustering for Metagame Analysis},
year = 2019
}%0 Conference Paper
%1 DocSch2019a
%A Dockhorn, Alexander
%A Schwensfeier, Tony
%A Kruse, Rudolf
%B Proceedings of the 11th Conference of the European Society for Fuzzy Logic and Technology (EUSFLAT 2019)
%D 2019
%P 536-543
%R 10.2991/eusflat-19.2019.74
%T Fuzzy Multiset Clustering for Metagame Analysis
%U https://www.atlantis-press.com/proceedings/eusflat-19/125914844
%@ 978-94-6252-770-6 - Dengel, R., Woiwode, D., Florsch{ü}tz, N., Huber, V., Muller, T., von Pichowski, J., Rabinowitsch, A., Scholz, S., Sch{ü}lein, H., Steinweg, E., Stippel, B., St{ö}ferle, P., Wittekind, I., Wizemann, O., Zaft, A., Zembrot, L., and Griebenow, K. (2019)QUEST ON BEXUS 27. In 24th ESA Symposium on European Rocket \& Balloon Programmes and Related.
@inproceedings{DenWoi2019,
author = {Dengel, Ric and Woiwode, Dominik and Florsch{ü}tz, Nico and Huber, Valentin and Muller, Tim and von Pichowski, Jan and Rabinowitsch, Alexander and Scholz, Sebastian and Sch{ü}lein, Hans and Steinweg, Eike and Stippel, Benjamin and St{ö}ferle, Peter and Wittekind, Isabell and Wizemann, Oliver and Zaft, Alexander and Zembrot, Lukas and Griebenow, Katrin},
booktitle = {24th ESA Symposium on European Rocket \& Balloon Programmes and Related},
keywords = {ON},
month = 10,
title = {QUEST ON BEXUS 27},
year = 2019
}%0 Conference Paper
%1 DenWoi2019
%A Dengel, Ric
%A Woiwode, Dominik
%A Florsch{ü}tz, Nico
%A Huber, Valentin
%A Muller, Tim
%A von Pichowski, Jan
%A Rabinowitsch, Alexander
%A Scholz, Sebastian
%A Sch{ü}lein, Hans
%A Steinweg, Eike
%A Stippel, Benjamin
%A St{ö}ferle, Peter
%A Wittekind, Isabell
%A Wizemann, Oliver
%A Zaft, Alexander
%A Zembrot, Lukas
%A Griebenow, Katrin
%B 24th ESA Symposium on European Rocket \& Balloon Programmes and Related
%D 2019
%T QUEST ON BEXUS 27 - Held, P., Dockhorn, A., and Kruse, R. (2014)On Merging and Dividing of Barabasi-Albert-graphs. In 2014 IEEE Symposium on Evolving and Autonomous Learning Systems (EALS).
@inproceedings{HelDoc2014,
author = {Held, Pascal and Dockhorn, Alexander and Kruse, Rudolf},
booktitle = {2014 IEEE Symposium on Evolving and Autonomous Learning Systems (EALS)},
keywords = {On},
title = {On Merging and Dividing of Barabasi-Albert-graphs},
volume = 444,
year = 2014
}%0 Conference Paper
%1 HelDoc2014
%A Held, Pascal
%A Dockhorn, Alexander
%A Kruse, Rudolf
%B 2014 IEEE Symposium on Evolving and Autonomous Learning Systems (EALS)
%D 2014
%R 10.1109/EALS.2014.7009499
%T On Merging and Dividing of Barabasi-Albert-graphs
%U https://ieeexplore.ieee.org/document/7009499
%V 444
%@ 978-1-4799-4494-1