
Research
Use Cases
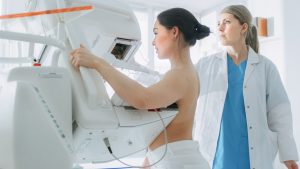
Team: Can Aykul, Jonas Wallat, Dr. Cameron Pierson, Prof. Dr. Maria-Esther Vidal
In the project "Breast Cancer Network Hannover", which focuses on breast cancer, Prof. Tjoung-Won Park-Simon and Dr. Thilo Dörk-Bousset from the Department of Gynaecology (MHH) are cooperating with the Leibniz AI Lab to identify factors for therapeutic success in patients diagnosed with breast cancer. For this purpose, standardized data of about 5000 patients of the regional network "Network Breast Cancer" will be analysed. In a first step, medical history data of the patient and her family, tumor characteristics, therapy data, data on follow-up examinations and survival, genetic information as well as socioeconomic data of the patient will be integrated to enable a comprehensive analysis. Special emphasis will be placed on the association of socioeconomic aspects such as education and migration background with therapeutic success. Another focus is on the identification of sub-populations of patients based on the success of different therapy options to enable targeted, personalized therapy. In particular, the project aims to give optimized suggestions which patients will benefit more from neo-adjuvant therapy and which patients will benefit more from surgery.
While the current approach to predict relapse probability is using a logistic regression model, we aim to expand to more involved models such as decision trees, random forests, neural networks and introducing existing domain knowledge on breast cancer using knowledge graphs. Hence, a knowledge graph will be modeled and populated based on obtained patient data. Building upon benchmark knowledge graph embedding models such as TransE [1], ComplEx [2] and RotatE [3] a framework that can incorporate existing biomedical ontologies (e.g. Gene Ontology) will be developed and thence relapse probability of a treatment will be predicted. On top of this, in order to assist decision making of the clinician, a drug-drug interaction knowledge graph will be used to learn latent semantic representations of drugs/medications to predict potentially harmful drug interactions that may occur if a patient is required to take multiple medications simultaneously. While introducing more complex models, we will need to balance model performance and interpretability of our approaches. Especially with the use of neural networks, we will use existing interpretability techniques such as LIME [4] and Shapley Values [5].
Given the ethical implications of developing and using machine learning models as healthcare decision support systems, we use this opportunity to evaluate an existing ethical framework in parallel to developing the solutions described above: The rapid and increasing development of machine learning in healthcare applications (ML-HCAs) requires ethical examination to assess the impact of novel medical devices and methods on patient and society. It is imperative that such ethical examinations are made to elucidate the associated ethical considerations, whether known or new. As medical technology advances so must the concurrent ethical examination of use and scope, such as the nature of system application, the data underwriting said system, and impacts to patient, society, and healthcare. Such ethical examination is imperative to avoid embedding or amplifying biases into machine learning tools used in healthcare.
While ethical frameworks have been proposed (e.g., Floridi & Strait, 2020; Saltz & Dewar, 2019), Char and colleagues (2020) develop a framework is thoroughly and clearly constructed from pre-existing literature to systematically identify ethical considerations specific to ML-HCAs. While some argue for an ‘ethicist-as-designer’ auditing the developmental process of machine learning tools (van Wynsberghe & Robbins, 2014), there is increased benefit of implementation of such an ethical identification framework with a research team. As has been suggested elsewhere (e.g, Armstrong, 2017; Blay et al., 2012), the development of AI in medicine ought to be interdisciplinary and/or by co-design. Therefore, implementation of Char and colleague’s (2020) framework with a research team provides the benefit of auditing (i.e., van Wynsberghe & Robbins, 2014) from the investigators of this study, while also promoting ethical consideration identification and management in situ of the research group. Such implementation would promote the ethical development of ML-HCAs. The proposed framework, however, has yet to be independently evaluated. Thus, we aim to evaluate Char and colleagues’ (2020) pipeline framework within the context of a research group seeking to develop machine learning techniques to identify biomarkers of breast cancer patients to predict patient success to chemotherapy treatment.
References:
[1] Bordes, Antoine, et al. "Translating embeddings for modeling multi-relational data." Advances in neural information processing systems 26 (2013). [2] Trouillon, Théo, et al. "Complex embeddings for simple link prediction." International conference on machine learning. PMLR, 2016. [3] Sun, Zhiqing, et al. "Rotate: Knowledge graph embedding by relational rotation in complex space." arXiv preprint arXiv:1902.10197 (2019). [4] M. Ribeiro - “Why Should I Trust You?” Explaining the Predictions of Any Classifier - https://dl.acm.org/doi/pdf/10.1145/2939672.2939778 [5] S. Lundberg - A Unified Approach to Interpreting Model Predictions - https://www.semanticscholar.org/paper/A-Unified-Approach-to-Interpreting-Model-Lundberg-Lee/442e10a3c6640ded9408622005e3c2a8906ce4c2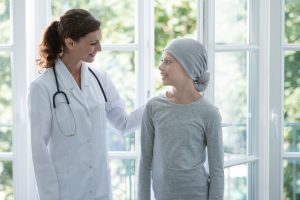
Team: Michelle Tang, PD Dr. Anke Bergmann
B-progenitor acute lymphoblastic
leukemia (B-ALL) is the most common pediatric malignancy. Next Generation Sequencing (NGS) technologies have been incorporated into routine diagnostics. Among them, the cost-effective targeted RNA sequencing is particularly appealing. We analyzed targeted RNA sequencing on ~1,500 pediatric ALL patients from the German pediatric ALL study groups. We combine UMAP (Uniform Manifold Approximation and Projection) and supervised machine learning algorithms to build an interactive tool for visualization and prediction of diagnostic subgroups. We explore a variety of machine learning techniques including gene network informed neural networks to build our predictive model. The tool helps to stratify patients without aberrant fusion or aneudiploidy, validate conventional diagnostic methods and discover new subgroups. In the future, we plan to expand such AI assisted diagnostic tool to more clinical , transcriptomic and epigenetic data. The proposed workflow will greatly complement the current diagnostic routine, provide better treatment options for patients and pave the way for personalized oncology.
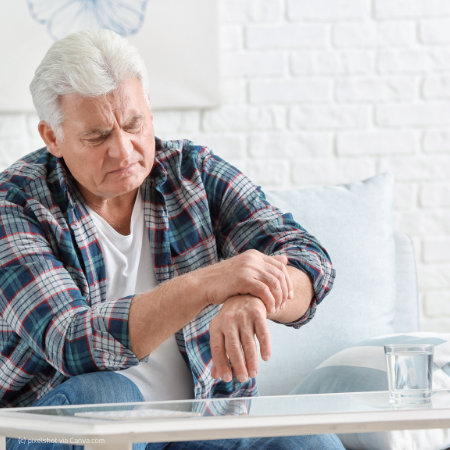
In the project "Big Data in Psychiatric Disorders", Prof. Dr. Helge Frieling of the Department of Psychiatry, Social Psychiatry and Psychotherapy (MHH) is working together with the Leibniz AI Lab on the focus areas of schizophrenia and neurodegenerative diseases. In the first sub-project, genetic information from around 50,000 patients diagnosed with schizophrenia is being evaluated using artificial intelligence in order to identify possible subtypes. The hypothesis here is that schizophrenia as a phenotype is based on a wide variety of causes that require differentiated diagnosis and therapy. We will focus on this project and have completed the data request formalities. However, we are yet to receive the data from NIMH.
Therefore, we are working on patient subtyping of Parkinson‘s disease, a neuro-degenerative disease, using clinical and genetic data. Most works focus of patient subtyping of Parkinson Disease (PD) based on motor symptoms and typically the population consider older population (above the age of 60 years). Recently, researchers also include non-motor symptoms to define patient subtypes because non-motor symptoms often precede the development of classical motor signs and contribute significantly to overall prognosis. Specifically, we plan to identify patient subtypes in younger patients with PD (below the age of 60 years) in terms of clinical and genetic data. We are also interested in patients with comorbodities like schizophrenia, severe depression. We have developed a binary classification model for predicting whether a patient has PD or not. We use the learnt decision tree to determine the patient subtypes; this is the first approach we take to overcome the limitation that the ground truth patient subtype labels are not available. Currently, we are performing a characterisation study of PD patient subtypes in terms of clinical data. In future, we plan to further characterize these clinical patient subtypes in terms of their genotype data. Along the same lines, we are currently exploring a second approach for patient subtyping where we directly cluster the patients in terms of their genotype data (SNP data).
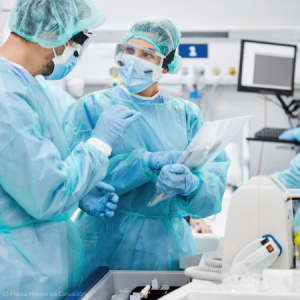
Team: Leonie Basso, Jingge Xiao, Seham Nasr, Dr. Zhao Ren, Prof. Antje Wulff, PD. Dr. Thomas Jack, PD. Dr. Henning Rathert, Marcel Mast, Prof. Michael Marschollek, Prof. Wolfgang Nejdl
In the project of “Pediatric Intensive Care Unit (PICU) use case”, Professor Antje Wulff, PD Dr. Thomas Jack, PD. Dr. Henning Rathert, Marcel Mast and Prof. Michael Marschollek from Hannover Medical School are working with the Leibniz AI Lab on the target of automatically detecting organ dysfunction in PICUs. Due to immediate decision-making with high risk and stress at a high level for clinicians in ICU wards, a data-intensive environment, it is essential to develop automatic decision-making models with the state-of-the-art machine learning and deep learning topologies; thus, promoting the development of real-time models for making decisions and mitigating the pressure of clinicians. More importantly, there are several difficulties during the decision-making procedure in PICUs: i) Different diseases dominate specific age groups from 0 to 18 years, and ii) normative values spread widely in different age groups. However, there are only a few research studies working on analysis of the data collected from PICU wards. In this regard, the project of PICU use case focuses on predicting organ dysfunction based on PICU data. There are two major branches that have been planned in this project. In the following, the two branches will be introduced.
i) We will focus on processing the clinical data which mainly contains vital signs (e.g., respiratory rate, heart rate, etc), laboratory parameters (e.g., leucocytes), and patient data (e.g., height, weight, etc).
ii) A new database of the waveform data (e.g., electrocardiogram) from the bedside monitors will be collected. The benchmark will be set up when the data is collected and pre-processed (e.g., anonymization) and a series of machine learning and deep learning approaches will be applied.
In summary, the research of this project is expected to facilitate related research studies in the applications of AI in PICU wards.COVID-19, a disease caused by SARS-CoV2, can take many different forms, ranging in clinical severity from mild or asymptomatic illness to acute conditions such as ARDS (acute respiratory distress syndrome) and death. Several studies have already shown that, in addition to demographic factors and pre-existing conditions, genetic predisposition may play an important role in disease development. To better understand the pathophysiology and progression of COVID-19, clinicians and researchers at Hannover Medical School (MHH) have been collecting patient samples and data in the COVID-19 Biobank funded by the Lower Saxony Ministry of Science and Culture (MWK) since the beginning of the pandemic.
Broad molecular characterizations have been performed on the collected biospecimens, particularly on material from patients with severe clinical courses requiring intensive care and respiratory support. These global analyses include sequencing of the patient genome, gene expression, and the methylation state of specific bases in the genome (epigenome). These data are complemented by high-resolution optical analyses of structural DNA variants that may be associated with increased disease risk. In addition, a broad clinical dataset on all patients was collected by the Hannover Unified Biobank (HUB) in collaboration with the Pneumology Department of the MHH, which includes information on COVID-19 patients' previous disease, disease severity, therapeutic measures, complications, and disease outcome.
To bring together this extensive collection of molecular and clinical data, already comprising over 14 TB in its raw state, in an integrative analysis, the HUB is collaborating with scientists from the L3S Future Laboratory and Prof. Yang Li from the Helmholtz Centre for Infection Research (HZI). The integrative data analysis aims to bring together the different data layers and identify prognostic molecular markers or early disease patterns associated with further disease progression.
Future Lab Seminars
Publications
Web APP
Our work “A message passing framework with multiple data integration
for miRNA-disease association prediction” has been published in Scientific Reports. (https://www.nature.com/articles/s41598-022-20529-5).
We provide a web application accompanying this work to make the results easily accessible, and to foster assessments and future adoption. Using the web application, you can query verified information as well as the predictions of our model for specific miRNAs, diseases or pathways, covering 1618 miRNAs and 3679 diseases.
Web application: http://software.mpm.leibniz-ai-lab.de/
Forschung
Anwendungsbereiche
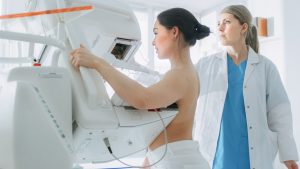
Team: Can Aykul, Jonas Wallat, Dr. Cameron Pierson, Prof. Dr. Maria-Esther Vidal
Im Projekt " Breast Cancer Network Hannover", das sich auf Brustkrebs konzentriert, arbeiten Prof. Tjoung-Won Park-Simon und Dr. Thilo Dörk-Bousset aus der Frauenklinik der MHH mit dem Leibniz AI Lab zusammen, um Faktoren für den Therapieerfolg bei Patientinnen mit der Diagnose Brustkrebs zu identifizieren. Dazu werden standardisierte Daten von rund 5000 Patientinnen des regionalen Netzwerks " Network Breast Cancer" ausgewertet. In einem ersten Schritt werden Anamnesedaten der Patientin und ihrer Familie, Tumormerkmale, Therapiedaten, Daten zu Nachuntersuchungen und Überleben, genetische Informationen sowie sozioökonomische Daten der Patientin integriert, um eine umfassende Analyse zu ermöglichen. Besonderes Augenmerk wird dabei auf die Assoziation von sozioökonomischen Aspekten wie Bildung und Migrationshintergrund mit dem Therapieerfolg gelegt. Ein weiterer Schwerpunkt ist die Identifizierung von Subpopulationen von Patienten auf der Grundlage des Erfolgs verschiedener Therapieoptionen, um eine gezielte, personalisierte Therapie zu ermöglichen. Insbesondere soll das Projekt optimierte Vorschläge liefern, welche Patienten eher von einer neoadjuvanten Therapie und welche eher von einer Operation profitieren werden.
Während der derzeitige Ansatz zur Vorhersage der Rückfallwahrscheinlichkeit ein logistisches Regressionsmodell verwendet, wollen wir auf komplexere Modelle wie decision trees (Entscheidungsbäume), random forests (Zufallswälder), neuronale Netze und die Einführung von vorhandenem Fachwissen über Brustkrebs unter Verwendung von Knowledge Graphs (Wissensgraphen) erweitern. Dazu wird ein Knowledge Graph modelliert und auf der Grundlage der erhaltenen Patientendaten ausgefüllt. Aufbauend auf Benchmark-Modellen zur Einbettung von Knowledge Graphs wie TransE [1], ComplEx [2] und RotatE [3] wird ein Rahmenwerk entwickelt, das bestehende biomedizinische Ontologien (z.B. Gene Ontology) einbeziehen kann, um so die Rückfallwahrscheinlichkeit einer Behandlung vorherzusagen. Darüber hinaus wird zur Unterstützung der Entscheidungsfindung des Arztes ein Knowledge Graph für Arzneimittelinteraktionen verwendet, um latente semantische Darstellungen von Arzneimitteln/Medikamenten zu erlernen und potenziell schädliche Arzneimittelinteraktionen vorherzusagen, die auftreten können, wenn ein Patient mehrere Medikamente gleichzeitig einnehmen muss. Bei der Einführung komplexerer Modelle müssen wir ein Gleichgewicht zwischen Modellleistung und Interpretierbarkeit unserer Ansätze finden. Insbesondere bei der Verwendung von neuronalen Netzen werden wir bestehende Interpretierbarkeitstechniken wie LIME [4] und Shapley-Werte [5] nutzen.
Angesichts der ethischen Implikationen der Entwicklung und Verwendung von Modellen des maschinellen Lernens als Entscheidungsunterstützungssysteme im Gesundheitswesen nutzen wir diese Gelegenheit, um parallel zur Entwicklung der oben beschriebenen Lösungen einen bestehenden ethischen Rahmen zu bewerten: Die rasche und zunehmende Entwicklung des maschinellen Lernens im Gesundheitswesen (ML-HCAs, englisch: machine learning in healthcare applications) erfordert eine ethische Prüfung, um die Auswirkungen neuartiger medizinischer Geräte und Methoden auf Patienten und Gesellschaft zu bewerten. Es ist zwingend erforderlich, dass solche ethischen Untersuchungen und die damit verbundenen ethischen Aspekte untersucht werden. In dem Maße, in dem die Medizintechnik voranschreitet, muss auch eine gleichzeitige ethische Prüfung der Nutzung und des Anwendungsbereichs erfolgen, z. B. der Art der Systemanwendung, der dem System zugrunde liegenden Daten und der Auswirkungen auf den Patienten, die Gesellschaft und das Gesundheitswesen. Eine solche ethische Prüfung ist zwingend erforderlich, um zu vermeiden, dass maschinelle Lernwerkzeuge, die im Gesundheitswesen eingesetzt werden, Verzerrungen enthalten oder verstärken.
Es wurden bereits ethische Rahmenwerke vorgeschlagen (z. B. Floridi & Strait, 2020; Saltz & Dewar, 2019), doch Char und Kollegen (2020) haben ein Rahmenwerk entwickelt, das sorgfältig und transparent auf der Grundlage bereits vorhandener Literatur aufgebaut ist, um systematisch ethische Überlegungen zu identifizieren, die für ML-HCAs spezifisch sind. Während einige für einen "Ethiker als Designer" plädieren, der den Entwicklungsprozess von Werkzeugen des maschinellen Lernens prüft (van Wynsberghe & Robbins, 2014), hat die Implementierung eines solchen ethischen Identifikationsrahmens in einem Forschungsteam einen größeren Nutzen. Wie bereits an anderer Stelle vorgeschlagen wurde (z. B. Armstrong, 2017; Blay et al., 2012), sollte die Entwicklung von KI in der Medizin interdisziplinär und/oder durch Co-Design erfolgen. Daher bietet die Umsetzung des Rahmens von Char und Kollegen (2020) in einem Forschungsteam den Vorteil der Überprüfung (z. B. van Wynsberghe & Robbins, 2014) durch die Forscher dieser Studie und fördert gleichzeitig die Identifizierung und das Management ethischer Überlegungen vor Ort in der Forschungsgruppe. Eine solche Umsetzung würde die ethische Entwicklung von ML-HCAs fördern. Der vorgeschlagene Rahmen muss jedoch noch unabhängig evaluiert werden. Daher wollen wir den Pipeline-Rahmen von Char und Kollegen (2020) im Kontext einer Forschungsgruppe bewerten, die maschinelle Lerntechniken zur Identifizierung von Biomarkern bei Brustkrebspatientinnen entwickeln will, um den Erfolg der Chemotherapie vorherzusagen.
Quellenangaben:
[1] Bordes, Antoine, et al. "Translating embeddings for modeling multi-relational data." Advances in neural information processing systems 26 (2013). [2] Trouillon, Théo, et al. "Complex embeddings for simple link prediction." International conference on machine learning. PMLR, 2016. [3] Sun, Zhiqing, et al. "Rotate: Knowledge graph embedding by relational rotation in complex space." arXiv preprint arXiv:1902.10197 (2019). [4] M. Ribeiro - “Why Should I Trust You?” Explaining the Predictions of Any Classifier - https://dl.acm.org/doi/pdf/10.1145/2939672.2939778 [5] S. Lundberg - A Unified Approach to Interpreting Model Predictions - https://www.semanticscholar.org/paper/A-Unified-Approach-to-Interpreting-Model-Lundberg-Lee/442e10a3c6640ded9408622005e3c2a8906ce4c2
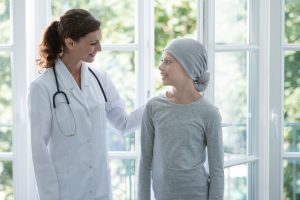
Team: Michelle Tang, PD Dr. Anke Bergmann
Die akute lymphoblastische Leukämie der B-Generationen (B-ALL, engl.: B-progenitor acute lymphoblastic leukemia) ist die häufigste pädiatrische Malignität. Next Generation Sequencing (NGS)-Technologien haben Einzug in die Routinediagnostik gehalten. Unter ihnen ist die kosteneffektive gezielte RNA-Sequenzierung besonders attraktiv. Wir analysierten die gezielte RNA-Sequenzierung von ~1.500 pädiatrischen ALL-Patienten aus den deutschen pädiatrischen ALL-Studiengruppen. Wir kombinieren UMAP (Uniform Manifold Approximation and Projection) und überwachte Algorithmen des maschinellen Lernens, um ein interaktives Tool zur Visualisierung und Vorhersage von diagnostischen Untergruppen zu entwickeln. Wir erforschen eine Vielzahl von maschinellen Lerntechniken, einschließlich über Gennetzwerke informierte neuronale Netze, um unser Vorhersagemodell zu erstellen. Das Tool hilft bei der Stratifizierung von Patienten ohne aberrante Fusion oder Aneudiploidie, bei der Validierung konventioneller diagnostischer Methoden und bei der Entdeckung neuer Untergruppen. Für die Zukunft planen wir, ein solches KI-gestütztes Diagnosewerkzeug auf weitere klinische, transkriptomische und epigenetische Daten auszuweiten. Der vorgeschlagene Arbeitsablauf wird die derzeitige diagnostische Routine erheblich ergänzen, den Patienten bessere Behandlungsmöglichkeiten bieten und den Weg für die personalisierte Onkologie ebnen.Accordion Sample Description
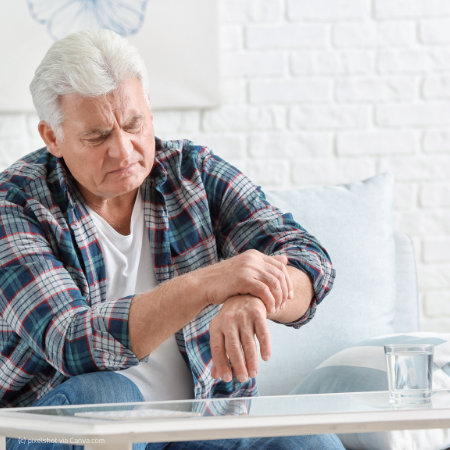
Team: Soumyadeep Roy, Salomon Kabongo Kabenamualu, Prof. Niloy Ganguly, Prof. Dr. Helge Frieling, Dr. Stefanie Mücke, Dominik Wolff
Im Projekt "Big Data in Psychiatric Disorders" arbeitet Prof. Dr. Helge Frieling von der Klinik für Psychiatrie, Sozialpsychiatrie und Psychotherapie (MHH) gemeinsam mit dem Leibniz AI Lab an den Schwerpunkten Schizophrenie und neurodegenerative Erkrankungen. Im ersten Teilprojekt werden genetische Informationen von rund 50.000 Patienten mit der Diagnose Schizophrenie mittels künstlicher Intelligenz ausgewertet, um mögliche Subtypen zu identifizieren. Die Hypothese dabei ist, dass Schizophrenie als Phänotyp auf einer Vielzahl von Ursachen beruht, die eine differenzierte Diagnose und Therapie erfordern. Wir werden uns auf dieses Projekt konzentrieren und haben die Formalitäten der Datenanforderung abgeschlossen. Allerdings stehen die Daten vom NIMH noch aus.
Daher arbeiten wir an der Subtypisierung von Patienten mit der Parkinson-Krankheit, einer neurodegenerativen Erkrankung, unter Verwendung klinischer und genetischer Daten. Die meisten Arbeiten befassen sich mit der Subtypisierung von Parkinson-Patienten anhand der motorischen Symptome und berücksichtigen in der Regel die ältere Bevölkerung (über 60 Jahre). In jüngster Zeit beziehen Forscher auch nicht-motorische Symptome in die Definition von Patientensubtypen ein, da nicht-motorische Symptome häufig der Entwicklung klassischer motorischer Anzeichen vorausgehen und wesentlich zur Gesamtprognose beitragen. Konkret planen wir, bei jüngeren Morbus-Parkinson-Patienten (unter 60 Jahren) anhand von klinischen und genetischen Daten Patientensubtypen zu identifizieren. Wir sind auch an Patienten mit Begleiterkrankungen wie Schizophrenie und schweren Depressionen interessiert. Wir haben ein binäres Klassifikationsmodell entwickelt, mit dem wir vorhersagen können, ob ein Patient an Morbus Parkinson leidet oder nicht. Wir verwenden den trainierten Entscheidungsbaum (decision tree), um die Patientensubtypen zu bestimmen; dies ist der erste Ansatz, den wir verfolgen, um die Einschränkung zu überwinden, dass die Subtypkennzeichnungen der Patienten nicht verfügbar sind. Derzeit führen wir eine Studie zur Charakterisierung der Subtypen von Parkinson-Patienten anhand klinischer Daten durch. In Zukunft planen wir, diese klinischen Patientensubtypen anhand ihrer Genotypdaten weiter zu charakterisieren. In diesem Sinne erforschen wir derzeit einen zweiten Ansatz für die Subtypisierung von Patienten, bei dem wir die Patienten direkt anhand ihrer Genotypdaten (SNP-Daten) clustern.
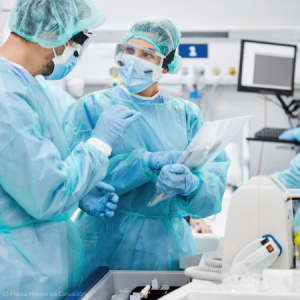
Team: Leonie Basso, Jingge Xiao, Seham Nasr, Dr. Zhao Ren, Prof. Antje Wulff, PD. Dr. Thomas Jack, PD. Dr. Henning Rathert, Marcel Mast, Prof. Michael Marschollek, Prof. Wolfgang Nejdl
Im Projekt "Anwendungsfall Pädiatrische Intensivstation (PICU, engl. Pediatric Intensive Care Unit)" haben Professorin Antje Wulff, PD Dr. Thomas Jack, PD. Dr. Henning Rathert, Marcel Mast und Prof. Michael Marschollek von der Medizinischen Hochschule Hannover gemeinsam mit dem Leibniz AI Lab an dem Ziel, Organdysfunktionen auf PICUs automatisch zu erkennen. Aufgrund der unmittelbaren Entscheidungsfindung mit hohem Risiko und hohem Stress für Kliniker auf Intensivstationen, einer datenintensiven Umgebung, ist es unerlässlich, automatische Entscheidungsfindungsmodelle mit dem neuesten Stand des maschinellen Lernens und der Deep-Learning-Topologien zu entwickeln; dadurch wird die Entwicklung von Echtzeitmodellen für die Entscheidungsfindung gefördert und der Druck auf die Kliniker gemindert. Darüber hinaus gibt es bei der Entscheidungsfindung in der PICU mehrere Schwierigkeiten: i) Verschiedene Krankheiten dominieren bestimmte Altersgruppen von 0 bis 18 Jahren, und ii) normative Werte sind in verschiedenen Altersgruppen sehr unterschiedlich. Es gibt jedoch nur wenige Forschungsstudien, die sich mit der Analyse der auf PICU-Stationen erhobenen Daten befassen. In diesem Zusammenhang konzentriert sich das Projekt PICU Use Case auf die Vorhersage von Organdysfunktionen auf der Grundlage von PICU-Daten. Es gibt zwei Hauptbereiche, die in diesem Projekt geplant sind. Im Folgenden werden die beiden Zweige vorgestellt.
i) Wir werden uns auf die Verarbeitung der klinischen Daten konzentrieren, die hauptsächlich Vitalparameter (z. B. Atemfrequenz, Herzfrequenz usw.), Laborparameter (z. B. Leukozyten) und Patientendaten (z. B. Größe, Gewicht usw.) enthalten.
ii) Es wird eine neue Datenbank mit Kurvenformdaten (z. B. Elektrokardiogramm) von den bettseitigen Monitoren erfasst. Der Benchmark wird eingerichtet, wenn die Daten gesammelt und vorverarbeitet sind ( zum Beispiel Anonymisierung), und es werden eine Reihe von Ansätzen des maschinellen Lernens und des Deep Learning angewendet.
Zusammenfassend lässt sich sagen, dass die Forschung im Rahmen dieses Projekts verwandte Forschungsstudien über die Anwendung von KI auf der Intensivstation erleichtern soll.
COVID-19, eine durch SARS-CoV2 verursachte Krankheit, kann viele verschiedene Formen annehmen, die in ihrem klinischen Schweregrad von leichten oder asymptomatischen Erkrankungen bis hin zu akuten Zuständen wie ARDS ("acute respiratory distress syndrome", d. h. akutes Atemnotsyndrom) und Tod reichen. Mehrere Studien haben bereits gezeigt, dass neben demografischen Faktoren und Vorerkrankungen auch eine genetische Veranlagung eine wichtige Rolle bei der Krankheitsentwicklung spielen kann. Um die Pathophysiologie und den Verlauf von COVID-19 besser zu verstehen, sammeln Kliniker und Forscher der Medizinischen Hochschule Hannover (MHH) seit Beginn der Pandemie Patientenproben und Daten in der vom Niedersächsischen Ministerium für Wissenschaft und Kultur (MWK) finanzierten COVID-19-Biobank.
An den gesammelten Bioproben wurden umfangreiche molekulare Charakterisierungen durchgeführt, insbesondere an Material von Patienten mit schweren Krankheitsverläufen, die intensivmedizinisch betreut und beatmet werden mussten. Zu diesen globalen Analysen gehören die Sequenzierung des Patientengenoms, die Genexpression und der Methylierungszustand bestimmter Basen im Genom (Epigenom). Ergänzt werden diese Daten durch hochauflösende optische Analysen struktureller DNA-Varianten, die mit einem erhöhten Krankheitsrisiko in Verbindung gebracht werden können. Darüber hinaus wurde von der Hannover Unified Biobank (HUB) in Zusammenarbeit mit der Abteilung Pneumologie der MHH ein breiter klinischer Datensatz zu allen Patienten erhoben, der Informationen über die Vorerkrankungen, den Schweregrad der Erkrankung, therapeutische Maßnahmen, Komplikationen und den Krankheitsverlauf der COVID-19-Patienten enthält.
Um diese umfangreiche Sammlung von molekularen und klinischen Daten, die im Rohzustand bereits über 14 TB umfasst, in einer integrativen Analyse zusammenzuführen, arbeitet die HUB mit Wissenschaftlern des L3S Future Laboratory und Prof. Yang Li vom Helmholtz-Zentrum für Infektionsforschung (HZI) zusammen. Ziel der integrativen Datenanalyse ist es, die verschiedenen Datenschichten zusammenzuführen und prognostische molekulare Marker oder frühe Krankheitsmuster zu identifizieren, die mit dem weiteren Krankheitsverlauf in Verbindung stehen.
Seminare
Publikationen
Webapplikation
Unsere Arbeit “A message passing framework with multiple data integration for miRNA-disease association prediction” wurde in Scientific Reports veröffentlicht. (https://www.nature.com/articles/s41598-022-20529-5).
Wir stellen eine Webanwendung zu dieser Arbeit zur Verfügung, um die Ergebnisse leicht zugänglich zu machen und die Bewertung und zukünftige Nutzung zu fördern. Mit der Webanwendung können Sie verifizierte Informationen sowie die Vorhersagen unseres Modells für bestimmte miRNAs, Krankheiten oder Pfade abfragen, die 1618 miRNAs und 3679 Krankheiten abdecken.
Webanwendung: http://software.mpm.leibniz-ai-lab.de/