Artificial Intelligence, Causality and Personalized Medicine (AICPM 2022)
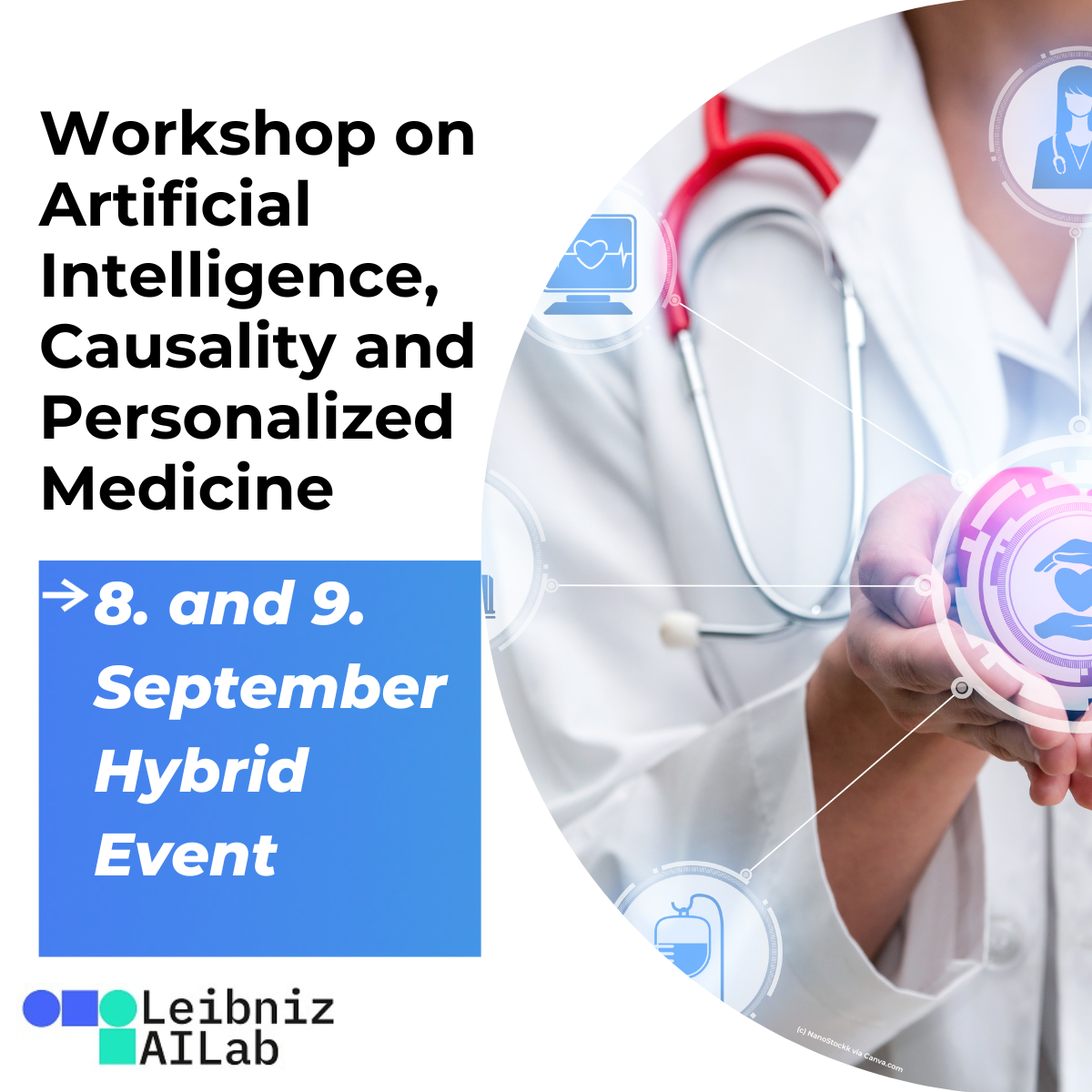
The AICPM workshop aims to bring together researchers interested in AI, Causality and Personalized Medicine, and move forward discussions on integrating causal reasoning into machine learning methods to tackle challenging AI problems in personalized medicine. We welcome researchers from all relevant disciplines, including but not limited to computer science, statistics, physics, philosophy, and medicine. Although personalized medicine is the primary focus application area for the workshop, researchers applying AI and Causality methods to other application areas (e.g., Earth Sciences, Finance etc.) are welcome.
Motivation
Machine learning methods have been immensely successful in tackling several problems across different domains such as computer vision, natural language processing, signal processing and many more. With the advent of deep neural networks, the performance of these methods have been pushed even further. However, in domains such as personalized medicine, increasingly it is being recognized that a fundamental piece is missing among these methods, which is causal reasoning. Cause and effect relationships are central in how we humans make sense of the world around us, how we act upon it, and how we respond to changes in our environment.
Current machine learning systems have no understanding of the relationship between causes and effects in their domain. As a result, they are brittle, cannot transfer to new domains, do not generalize except from one data point to the next (sampled from the same distribution) and they cannot explain their actions to users. Consequently, a new research direction has emerged: Integrating causality into machine learning methods, to pave the way for designing next generation intelligent systems. Given their causal reasoning, these methods will be suitable to tackle the issues affecting current methods such as explainability, generalizability and robustness, and allow researchers to represent medical background knowledge in an appropriate way.
Register here
Venue
The workshop will be held in a hybrid way, taking place at the Leibniz AI Lab & L3S Research Center, Leibniz Universität Hannover, Germany as well as online.
Speakers
Avishek Anand, TU Delft and L3S
Gjergji Kasneci, University of Tübingen
Marco Zaffalon, IDSIA and Artificialy
Philip Dawid, University of Cambridge
Prasenjit Mitra, Penn State University and L3S
Roi Reichert, Technion – Israel Institute of Technology
Tim Friede, Universitätsmedizin Göttingen
Ricardo Silva, University College London
Andreas Gerhardus, German Aerospace Center
Gourab Patro, IIT Kharagpur and L3S
Jalal Etesami, EPFL
Amit Sharma, Microsoft Research
Jonas Peters, University of Copenhagen
Stefan Bauer, KTH Stockholm
Zhiliang Wu, Siemens Technology
Sara Magliacane, University of Amsterdam and MIT-IBM Watson AI lab
Tom Claassen, Radboud University
Schedule
Day 1
Time | Speaker | Title | Presentations |
---|---|---|---|
09:00 - 09:15 | Opening and Welcome | ||
09:15 - 10:00 | Philip Dawid | What Should be the Focus of Statistical Personalized Medicine | Philip Dawid |
10:00 - 10:45 | Stefan Bauer | Towards Learning Causal Representations & Interactive Benchmarks | |
10:45 - 10:55 | Break | ||
10:55 - 11:30 | Sara Magliacane | Causality-inspired ML: what can causality do for ML? The domain adaptation case | Sara Magliacane |
11:30 - 12:15 | Jonas Peters | Exploiting Invariance: from Causal Discovery to Robust Decision Making | Jonas Peters |
12:15 - 13:25 | Lunch Break | ||
13:25 - 14:10 | Jalal Etesami | Foundations of Causal Inference: Challenges and Opportunities | |
14:10 - 14:45 | Andreas Gerhardus | Reliable causal discovery in time series | Andreas Gerhardus |
14:45 - 15:00 | Break | ||
15:00 - 15:45 | Gjergji Kasneci | Towards realistic and robust counterfactual explanations for tabular data | Gjergji Kasneci |
15:45 - 16:20 | Gourab Patro | Fair ranking: a critical review, challenges, and an impact-oriented research agenda for future | Gourab Patro |
16:20 - 16:55 | Roi Reichart | Causal models for NLP applications | Roi Reichart |
Day 2
Time | Speaker | Title | Presentation |
---|---|---|---|
09:00 - 09:45 | Amit Sharma | Causal Machine Learning: A necessary ingredient for building generalizable models | Amit Sharma |
09:45 - 10:30 | Marco Zaffalon | Causal EM for counterfactual inference, with an application to palliative care. | Marco Zaffalon |
10:30 - 10:40 | Break | ||
10:40 - 11:15 | Tom Claassen | Causal discovery from medical data: challenges and opportunities | Tom Claassen |
11:15 - 11:50 | Avishek Anand | Explainable Information Retrieval | |
11:50 - 13:00 | Lunch Break | ||
13:00 - 13:45 | Prasenjit Mitra | Adapting Tools of Causality to Analyze the Obesity Paradox | Prasenjit Mitra |
13:45 - 14:30 | Tim Friede | AI/ML in Clinical research | Tim Friede |
14:30 - 14:45 | Break | ||
14:45 - 15:30 | Ricardo Silva | Causes with many moving parts | Ricardo Silva |
15:30 - 16:15 | Zhiliang Wu | Learning Individualized Treatment Rules with Estimated Translated Inverse Propensity Score | Zhilliang Wu |
16:15 - 16:30 | Closing |